
Lektsii (1) / Lecture 12
.pdf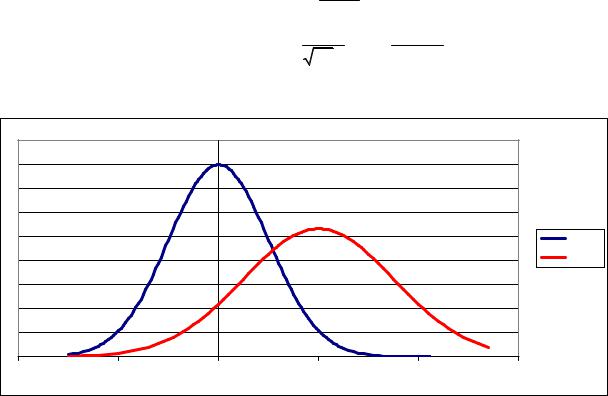
ICEF, 2012/2013 STATISTICS 1 year LECTURES
Lecture 12 |
27.11.12 |
GENERAL NORMAL DISTRIBUTION
Example. The histogram for the men’s’ weights looks like a standard normal but the peak is shifted to the point 72 kg, i.e. the mean is 72 kg and the standard deviation is 10 kg. How to find the proportion of people with the weight greater than 80 kg?
Z−score: Z = 80 −72 |
= 0.8, Pr(X >80) = Pr(Z > 0.8) =1−0.79 = 0.21 = 21% . |
|||||||
|
10 |
|
|
|
|
|
|
|
Let Z be a standard normal random variable, Z N (0,1) . Recall, that |
|
|
||||||
E(Z ) = µZ |
= 0, V (Z ) =σZ2 |
=1, σZ =1. Let µ, σ > 0 be two constants. Then |
||||||
|
|
|
X N (µ,σ) Z = |
X −µ |
N (0,1), |
|
|
|
|
|
|
|
σ |
|
|
|
|
|
|
p.d. f . for X : fX (x) = |
1 |
|
(x −µ)2 |
, |
||
|
|
πσ exp − |
2σ2 |
|
||||
|
|
|
2 |
|
|
|
|
|
|
|
|
E(X ) = µ, V (X ) =σ2 . |
|
|
|
||
|
|
|
0.45 |
|
|
|
|
|
|
|
|
0.4 |
|
|
|
|
|
|
|
|
0.35 |
|
|
|
|
|
|
|
|
0.3 |
|
|
|
|
|
|
|
|
0.25 |
|
|
|
|
phi(x) |
|
|
|
0.2 |
|
|
|
|
f(x) |
|
|
|
0.15 |
|
|
|
|
|
|
|
|
0.1 |
|
|
|
|
|
|
|
|
0.05 |
|
|
|
|
|
|
|
|
0 |
|
|
|
|
|
-4 |
-2 |
|
0 |
2 |
|
4 |
|
6 |
Example. The lifetime in some region has the normal distribution with the expectation 65 years and standard deviation 15 years. What is the proportion of population older than 75 years?
Let X be the lifetime of randomly selected man from the population. Then
X −65 |
|
10 |
|
|
|||
Pr(X > 75) = Pr |
|
> |
|
|
|
= Pr(Z > 0.667) =1−0.75 = 0.25 . |
|
15 |
15 |
||||||
|
|
|
|
JOINT DISTRIBUTION (совместное распределение)
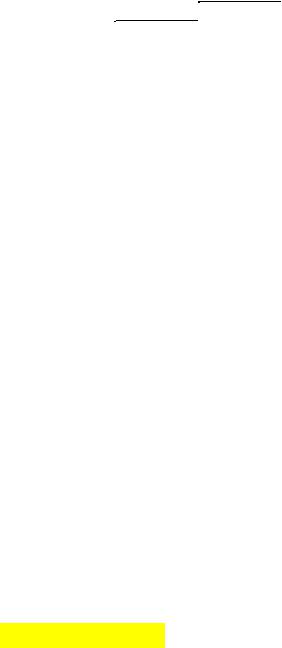
Consider the following example. Let X be the level of education of randomly selected person from some population, and Y be her/his monthly income. For simplicity we divide both education and income into some levels:
X = level of education |
|
|
||
1 |
− middle school |
2 − bachelor |
3 − master |
4 − PhD |
Y = monthly income |
|
|
|
|
1 |
− <500 |
2 − 500-1000 |
3 − 1000-1500 |
4 − 1500-2000 |
5 |
− >2000 |
|
|
|
The table below gives the join distribution of these two variables.
|
|
|
Y |
|
|
5 |
|
X |
1 |
2 |
3 |
4 |
Marginal |
||
1 |
0.1 |
0.06 |
0.04 |
|
0.02 |
0.01 |
0.23 |
2 |
0.08 |
0.09 |
0.07 |
0.03 |
0.05 |
0.32 |
|
3 |
0.04 |
0.1 |
0.08 |
0.06 |
0.06 |
0.34 |
|
4 |
0.01 |
0.02 |
0.04 |
0.02 |
0.02 |
0.11 |
|
Marginal |
0.23 |
0.27 |
0.23 |
0.13 |
0.14 |
1 |
For example, Pr(X = 2 ∩Y =1) = 0.08 . This may be interpreted in other way: the proportion of
those people in a population having, for example, 3d level of education and 2nd income level is 0.1.
GENERAL CASE
Let two random variables X and Y be given. The first has values x1,..., xm , while the second has values y1,..., yn . The probabilities of events (X = xi ) ∩(Y = y j ), i =1,..., m; j =1,..., n are given in the table below.
|
|
|
|
Y |
|
|
|
|
|
|
y1 |
y2 |
|
y j |
|
yn |
Margin X |
|
x1 |
p11 |
p12 |
|
p1 j |
|
p1n |
p1• |
|
x2 |
p21 |
p22 |
|
p2 j |
|
p2n |
p2• |
X |
|
|
|
|
|
|
|
|
|
xi |
pi1 |
pi2 |
|
pij |
|
pin |
pi• |
|
|
|
|
|
|
|
|
|
|
xm |
pm1 |
pm2 |
|
pmj |
|
pmn |
pm• |
|
Margin Y |
p•1 |
p•2 |
|
p• j |
|
p•n |
|
The set of these probabilities is called the Joint distribution of the random variables X and Y:
pij = Pr(X = xi ∩Y = y j ) .
Obviously any distribution satisfies the following conditions:
|
m |
n |
1) pij ≥ 0, i =1,..., m; j =1,..., n |
2) ∑∑pij =1. |
|
|
i=1 |
j=1 |
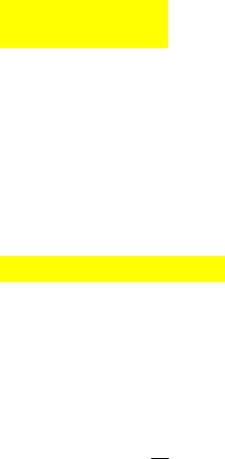
The individual distribution of each component X or Y is called Marginal distribution of the corresponding component.
Obviously, marginal distributions can be calculated as follows:
n |
m |
Pr(X = xi ) = pi• = ∑pij , |
Pr(Y = y j ) = p• j = ∑pij . |
j=1 |
i=1 |
So if the joint distribution is known the marginal distributions can be constructed, but not vise versa: knowing only marginal distributions one cannot reconstruct the joint distribution.
CONDITIONAL DISTRIBUTION AND CONDITIONAL EXPECTATION
Содержательно условное распределение описывает распределение одной из компонент в какой-либо части популяции, которая соответствует определённому значению второй компоненты. Возвращаясь к начальному примеру, можно задать естественный вопрос: как распределён доход в группе людей, имеющих, скажем, бакалаврское образование.
Formally, conditional distribution of Y given |
X = xi is defined as follows: |
|||||||||
p |
j|i |
= Pr(Y = y |
j |
| X = x ) = |
Pr((Y = y j ) ∩(X = xi )) |
= |
pij |
, j =1,..., n . |
|
|
|
|
|
||||||||
|
|
i |
Pr(X = xi ) |
|
|
pii |
|
|||
|
|
|
|
|
|
|
|
It should be emphasized that this is the distribution of the second component.
The expectation of this distribution, i.e. the number
E(Y | X = xi ) = ∑n y j p j|i
j=1
is called the conditional expectation of Y given X = xi .
Note that there are m different conditional expectations, one for each level x1,..., xm .
Non-formally the conditional expectation is the mean value of the component Y within the subpopulation with the given value of X.
INDEPENDENCE
The random variables Y and X are called independent if the events (X = xi ) and (Y = y j ) are independent for all xi , y j . That is Pr((X = xi ) ∩(Y = y j )) = Pr(X = xi ) Pr(Y = y j ) , or
equivalently,
pij = pii pi j , i =1,..., m; j =1,..., n .
Note that if X and Y are independent random variables the join distribution can be reconstructed by the marginal distributions.
Example. Let two fair dice (red and white) are thrown. Denote X the number of points on the red die and Y the number of points on the white die. Then Pr(X =i) = 16 , i =1,...,6 and
Pr(Y =i) = 16 , j =1,...,6 , and
Pr((X =i) ∩(Y = j)) = 361 = 16 16 = Pr(X =i) Pr(Y = j) . So, X and Y are independent, that is compatible with the intuition.