
Lektsii (1) / Lecture 17
.pdf
ICEF, 2012/2013 STATISTICS 1 year LECTURES
Лекция 17 |
22.01.13 |
Reminder. The distribution of the random variable |
|
t = (x −µ) |
n . |
s |
|
is called t−distribution or Student’s distribution with n−1 degrees of freedom. It can be proved (the corresponding result is called Fisher’s Lemma) that the distribution of t does not depend on parameters µ,σ and depends only on n (sample size). We will denote the t−distribution with k
degrees of freedom by t(k) . Thus
(x −µ) n t(n −1) . s
The pdf of t(k) is symmetric with respect to 0 and bell-shaped curve for each k. The
t(k) −distribution has “more heavy tails” in comparison with the standard normal distribution. As k increases the pdf of t(k) tends to pdf of standard normal distribution.
Since t(k) −distribution depends only on degrees of freedom it can be tabulated for each value of k =1, 2,.... Particularly, the α -percent points is tabulated for
α = 0.1, α = 0.05, α = 0.025, α = 0.01 and for n =1,...,100 . We have
|
|
|
(x −µ) |
n |
|
<t |
|
|
=1−α . |
|
|
||||||||||
|
|
|
|
||||||||||||||||||
|
Pr |
|
|
|
|
|
|
|
(n −1) |
|
|
||||||||||
|
|
|
|
s |
|
|
|
|
|
|
|
α / 2 |
|
|
|
|
|
|
|
|
|
|
|
|
|
|
|
|
|
|
|
|
|
|
|
|
|
|
|
|
|
|
|
|
|
|
|
|
|
|
|
|
|
|
|
|
|
|
|
|
|||||
Solving the inequality with respect to µ we get: |
|
|
|||||||||||||||||||
|
Pr x −tα / 2 (n −1) |
|
|
s |
|
< µ < x |
+tα / 2 (n −1) |
s |
=1−α . |
||||||||||||
|
|
n |
|
||||||||||||||||||
|
|
|
|
|
|
|
|
|
|
|
|
|
|
|
|
n |
|||||
This means that the interval |
|
|
|
|
|
|
|
|
|||||||||||||
|
x −tα / 2 |
(n −1) |
|
|
s |
; x |
+tα / 2 (n |
−1) |
s |
|
|
|
|
||||||||
|
|
|
n |
|
|
|
|
||||||||||||||
|
|
|
|
|
|
|
|
|
|
|
|
n |
|
|
|
||||||
is 100(1 |
−α)% −confidence interval for population mean µ . |
||||||||||||||||||||
Equivalently, |
|
|
|
|
|
|
|
|
|
|
|
|
|
|
|
|
|
|
|||
|
µ = x ±t |
(n −1) |
s |
. |
|
|
|
|
|
|
|
||||||||||
|
|
|
|
|
|
|
|
|
|||||||||||||
|
|
|
|
α / 2 |
|
|
|
|
|
|
|
n |
|
|
|
|
|
|
|
|
|
|
|
|
|
|
|
|
|
|
|
|
|
|
s =3.2 . Then t0.025 (19) = 2.093, and |
||||||||
Example. Let n = 20, x =98, |
|||||||||||||||||||||
µ =98 ±2.093 |
3.2 |
=98 |
±1.498 . |
|
|
|
|
|
|
||||||||||||
20 |
|
|
|
|
|
|
|||||||||||||||
|
|
|
|
|
|
|
|
|
|
|
|
|
|
|
|
|
|
|
|
Confidence interval for a difference of population means (independent samples)
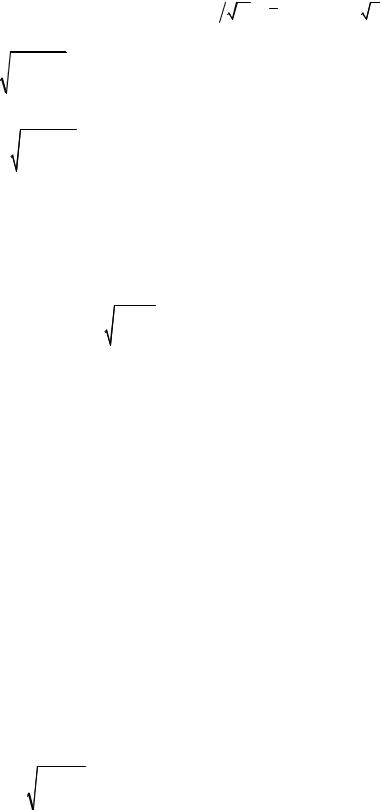
Quite often we have to compare the population means of two populations, e.g. mean incomes in two regions, mean life-times of identical details produced by different enterprises and so on. Thus we have to construct the confidence intervals for the difference of two population means.
More formally, let X N (µ1,σ1 ), Y N (µ2 ,σ2 ) be two independent normal populations. Let x1,..., xm and y1,..., yn be two samples from the corresponding populations. (Note that sample sizes may be different.) Then x N (µ1,σ1 m), y N (µ2 ,σ2 n) and due to independency of x, y we get:
|
µ1 |
−µ2 , |
σ2 |
+ |
σ2 |
|
x − y N |
1 |
2 |
. |
|||
|
|
|
m |
|
n |
|
|
|
|
|
|
|
|
Similar to the previous case we get the following 100(1−α)% −confidence interval:
µ |
−µ |
|
= x − y ± z |
σ2 |
σ2 |
(3) |
2 |
1 + |
2 . |
||||
1 |
|
α / 2 |
m |
n |
|
|
|
|
|
|
|
Obviously this formula can be used only in the non-realistic case when the standard deviations σ1, σ2 are known. If this is not the case we should use the estimates of these standard deviations
and change the distribution.
|
I. Standard deviations are unknown and |
σ1 =σ2 |
, exact confidence intervals. |
|
|
||||||||||||||||||||
If σ1 =σ2 |
≡σ then the formula (3) is modified as |
follows |
(it may be rigorously proved using |
||||||||||||||||||||||
again Fisher’s Lemma): |
|
|
|
|
|
|
|
|
|
|
|
|
|
|
|
|
|
|
|
|
|||||
|
µ −µ |
|
= x − y ±t |
|
(m +n −2) s |
p |
|
|
1 |
|
+ 1 |
|
|
|
|
(4) |
|
|
|
|
|||||
|
2 |
|
|
|
|
|
|
|
|
|
|
|
|
||||||||||||
|
1 |
|
|
α / 2 |
|
|
|
m |
n |
|
|
|
|
|
|
|
|
|
|
||||||
where |
|
|
|
|
|
|
|
|
|
|
|
|
|
|
|
|
|
|
|
|
|||||
|
|
m |
|
n |
|
|
|
|
|
|
|
|
|
|
|
|
|
|
|
|
|
|
|
||
|
|
|
|
|
|
|
|
|
|
|
|
|
|
|
|
|
|
|
|
|
|
|
|||
|
|
|
∑(xi − x )2 +∑( y j − y)2 |
|
(m −1) |
s |
2 |
+(n −1) |
s |
2 |
|
|
|
|
|
||||||||||
|
s2p = |
i=1 |
|
j=1 |
= |
x |
y |
|
|
|
|
||||||||||||||
|
|
|
|
|
|
|
|
|
|
|
|
|
|
|
|
||||||||||
|
|
m +n −2 |
|
|
|
|
m +n −2 |
|
|
|
|
|
|
|
|||||||||||
|
|
|
|
|
|
|
|
|
|
|
|
|
|
|
|
|
|||||||||
is the (unbiased) estimator of the variance σ2 |
by using the pooled sample x ,..., x |
; y ,..., y |
n |
. |
|||||||||||||||||||||
|
|
|
|
|
|
|
|
|
|
|
|
|
|
|
|
|
|
|
|
1 |
m |
1 |
|
Exercise. Prove that E(s2p ) =σ2 .
Example 1. Let X be a population salary in Moscow, and Y be a population salary in S.Petersburg. Sample mean salary of m = 60 randomly selected people in Moscow is x = 27450 and standard deviation is sx =3240 . For S.Petersburg we have n =50, y = 24650, sy = 2980 .
Assuming normal distributions of the salaries in both cities and the equality of standard deviations find 95%−confidence interval for the difference of population mean salaries.
Use formula (4). By direct calculations we get sp =3125 , t0.025 (108) =1.98 (using Excel), and
µ1 −µ2 = 2800 ±1187 .
Note that this interval does not contain 0. This give us statistical evidence in favor of the statement that the salaries in Moscow in average are greater than in S.Petersburg.
II. Standard deviations are unknown and arbitrary, approximate confidence intervals.
In this case formula (3) is simply modified as
|
|
= x − y ±t |
(k) |
s2 |
sy2 |
, k = min(m, n) −1 |
. |
(5) |
|
µ −µ |
2 |
x |
+ |
|
|||||
|
|
||||||||
1 |
α / 2 |
|
m |
n |
|
|
|
||
|
|
|
|
|
|
|
But this is an approximate confidence interval, and formula (5) can be used for sufficiently large values m, n .
Example 2. Using data from Example 1 find the 95%−confidence interval (5). Now t0.025 (49) = 2.01 and
µ1 −µ2 = 2800 ±1193.