
Jankowitcz D. - Easy Guide to Repertory Grids (2004)(en)
.pdf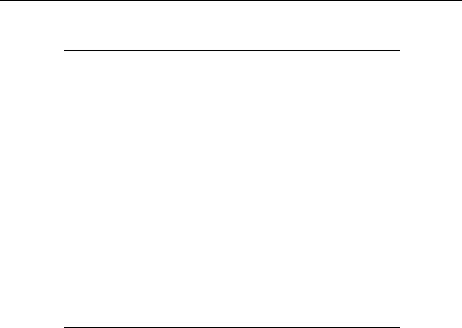
ANALYSING MORE THAN ONE GRID 169
Table 7.7 Categories summarising factors assessed in venture capital decision-making
Constructs |
No. |
|
|
Management |
|
Personal traits of the proposer |
|
Experience of the proposer |
|
Management team characteristics |
22 |
Continuity of the company/market |
|
Unique opportunity |
|
Product-market uniqueness |
9 |
Appropriate return |
|
Funding base and risk |
5 |
Other |
|
The proposal itself |
|
Use of technology |
9 |
Market location |
|
Total |
45 |
Source: Reproduced by permission Hisrich & Jankowicz (1990).
than relying entirely on the pre-existing analytic framework, is indicated by the fact that nine of the constructs could not be accommodated within the preexisting scheme. The nature of the proposal itself, the way in which it uses technology, and the geographical location (local versus distributed) also play a part, in ways which the pre-existing category scheme did not allow for.
7.3 HONEY’S CONTENT ANALYSIS
We seem to have solved the problem outlined at the start of this chapter: how to say something about the different meanings being expressed in a set of grids, making general statements about the sample as a whole, while reflecting the particular meanings being offered by individual interviewees as they express their personal and, at times, idiosyncratic knowledge.
But we’ve done so at a cost. We haven’t been able to use any of the ratings of elements on constructs available in the original grids. Somewhere in the whole set, we’ve retained each person’s constructs; but we’ve lost what each person was telling us about the topic by means of those personal constructs. This is the price we’ve paid for starting with individuals’ constructs, rather than using a standard set of constructs supplied to all the interviewees on which

170 THE EASY GUIDE TO REPERTORY GRIDS
ratings could be taken, totalled, averaged, and so on. We’ve lost a considerable amount of the information available in each grid.
7.3.1 Rationale
However, there is a technique, first developed by Honey (1979), which achieves what, at first sight, appears to be impossible. It aggregates different constructs across a sample and provides a way in which we can make use of some of the individual meanings being conveyed by each person’s ratings.
The technique assumes that what we’re interested in is each individual’s personal understanding of the topic in question, and treats each construct offered by the individual as more closely related, or less closely related, to the overall issue s/he has in mind when thinking about the topic.
This makes a lot of sense. Kelly’s theory asserts that constructs are organised into a system, with some constructs being superordinate to others (the Organisation Corollary), and, you’ll recall, we make use of this property when we seek to elicit more specific constructs by laddering downwards (see Section 4.4.1). Some constructs are crucial and central to the individual’s knowledge and views about the topic, while others, while relevant, are somewhat more peripheral. Moreover, we expect some kind of consistency among the constructs a person uses. So, for example, if an interviewee were to characterise an individual as‘unreliable’and at the same time ‘dependable’, we would be surprised, and look for a rationale. We expect constructs to hang together, to express a coherent point of view, and to tell a consistent story.
But Kelly also mentions that the different ways we have of making sense of different situations may not necessarily be compatible with each another (Fragmentation Corollary). A person’s construct system isn’t a monolithic structure. And so it is very reasonable to expect that some constructs are particularly related to any issue being construed, carrying more meaning about the topic in question. Others, while relevant, might flesh out the meaning, adding grace notes, as it were, without being ‘what the whole thing is really all about’.
In Honey’s technique, ‘what the individual has in mind when thinking about the topic’ is summarised by supplying a construct: an ‘overall summary’ construct designed to sum up the interviewee’s individual stance to the topic as a whole. (See Section 4.2.7 on supplied constructs and their uses. Refresh your memory now, before reading on!)
Thus, a grid designed to capture the reactions of a set of trainees to the different sessions of a course which they attended, in order to make improvements in the course, might involve a supplied construct which assessed the extent to which they felt they learnt: ‘overall, learnt a lot – overall, didn’t learn much’. A grid which assessed the knowledge in a company’s sales

ANALYSING MORE THAN ONE GRID 171
team about which approaches worked best in selling to their customers might conclude by asking each salesperson to rate each approach on ‘overall, more effective – overall, less effective’. A grid about personal friendship using the names of friends and acquaintances as the elements might require the interviewee to rate all of them on the supplied construct, ‘overall, a closer relationship – overall, a more distant relationship’. Table 7.8 provides some further examples.
Honey’s content analysis aggregates constructs across the sample, as in Section 7.2.1, but labels each construct with two indices reflecting the extent to which the ratings on the particular construct match the ratings on the ‘overall’ construct. The first index is our familiar % similarity score, as outlined in Section 6.1.2. One hundred per cent means that the ratings on the individual construct are identical to the ratings on the overall construct; 70% means that the ratings are less similar, and so on.
The second index reflects the fact that people differ in their typical % similarity scores. When we compare the ratings elicited from one interviewee on all of the constructs in the grid, we may find that they typically reveal a relatively narrow range of % similarity scores. The highest may be 100% and the lowest perhaps 80%. A second person, however, might be inclined to see many different, and relatively unrelated, aspects of the issue when thinking about the topic, and so her highest % similarity score may be 85% and the lowest, 55%. To the extent that different people have different ranges of % similarity scores for any topic, we can talk of different personal metrics. At 85%, what feels very similar for the second person may lie towards the bottom of the range of similarity for the first person.
And so, Honey’s procedure acknowledges that % similarity scores are relative, and as well as noting their actual percentage value, notes whether that value is placed among the high, the intermediate or the low (H-I-L) values (what Honey calls the ‘top-and-tail data’ for that particular individual.
Okay. So, taking both indices (% similarity and the H-I-L index) into account (see below for the detail), it’s clear that some constructs will match highly with the supplied ‘overall’ construct. In other words, they will represent what that particular individual felt and thought, overall, very well. Others, however, will match less highly with the ‘overall’ construct; that is, they will represent what the individual felt and thought about the topic, overall, somewhat less strongly.
The aggregated set of constructs for the sample as a whole will, in other words, represent the categorised views of all the individuals in the sample, but will also preserve information about each individual’s views in terms of how he or she severally, personally, idiosyncratically if you will, thought about the topic.

Table 7.8 Examples of ‘overall’ constructs in Honey’s content-analysis technique
|
|
Possible qualifying phrase |
Supplied construct |
Topic |
Elements |
‘. . . from the point of view of . . .’ |
‘overall . . .’ |
Lecturer effectiveness |
Lecturers, rated by a sample |
|
of students |
Counselling style |
Different types of ‘helper’: |
|
‘a friend’, ‘a counsellor’, |
|
‘a parent’, ‘a priest’ |
Being an effective clerical |
Different clerical officers, |
officer |
including some more |
|
effective and some less |
Understanding creative block |
Several of their own paintings |
in the figurative arts |
as nominated by each artist |
Thinking about what |
People you know, ranging |
friendship means to you |
from ‘best friend’ to |
|
‘disliked acquaintance’ |
. . . What they do that makes them more, or less, effective as lecturers
. . . What they do and how they do it that makes them more, or less, approachable to a person seeking help
. . . How they do their job which makes them good at it, or less good at it
. . .What was going on which made them easy to paint, or led to getting ‘stuck’
. . .What it is about them that makes for friendship – or otherwise!
. . . More effective – less effective
. . . More approachable – less approachable
. . . More effective clerical officer – less effective clerical officer
. . . More straightforward – more problematic
. . . A real friend – rather less so
1.In each case, your analysis will identify the constructs, in their categories, which each interviewee has particularly in mind when thinking about the issue involved in the topic, as that issue is summarised by the ‘overall’ construct. Thereby specifying what ‘effectiveness’, ‘approachability’, and so on mean in particular to him or her.
2.Notice how the ‘overall’ construct summarises the issue at stake, as previously highlighted by the qualifying phrase. (Glance again at the rationale for the use of qualifying phrases in Sections 3.2.1 and 3.2.3. Table 3.2 is worth another look too.)

ANALYSING MORE THAN ONE GRID 173
And it will take into account both the % similarity value and the individual’s personal metric!
This is tremendous. It’s pure gold. I have taken my hat off to Peter Honey for a quarter-century now, and shall continue to do so for another 50 years at least. I hope you can see why. While strongly advocating procedures for ensuring reliability, he doesn’t go into reliability measurement in any great detail; I have added that, below, together with some grace notes of my own. But the overall thrust is his; he gives us exactly what we need when we seek to aggregate large samples of respondents.
7.3.2 Procedure
The procedure is similar to the bootstrapping one outlined in Section 7.2.1, and looks as follows. You may find a glance at Figure 7.1 is helpful as you go through the steps below. It’s a grid completed by one of our sales staff in the publisher’s example shown in Tables 7.2 to 7.6.
(1)Obtain ratings on a supplied ‘overall’ construct. Make sure, when you elicit each grid, that you supply a construct which serves to sum up the interviewee’s overall stance on the topic. Ask the interviewee to rate all the elements on this supplied construct, as well as on the elicited constructs.
(2)Compute sums of differences for each construct against the ‘overall’ construct. Use the procedure defined in Section 6.1.2, ‘simple relationships between constructs’. Note: you’re doing this for every construct against the ‘overall’ construct only. For the purposes of our present analysis, you don’t have to work it out between each construct and each other construct. Bearing in mind that you have to check for reversals (that you might get a smaller sum of differences between a given construct and the ‘overall’ construct if one of the two is reversed), the quickest way of completing this step is to compute the sum of differences
(a)between ‘overall’ construct and the first construct
(b)between ‘overall’ construct (reversed) and the first construct (unreversed, of course)
(c)note the smaller of the two sums of differences.
Repeat for the ‘overall’ construct and each of the other constructs.
Doing it this way makes sure that you only have to reverse one set of ratings, those for the ‘overall’ construct; and, in fact, this part of the procedure is much quicker to do than to describe! (Glance at the very bottom row of ratings in Figure 7.1).

174 THE EASY GUIDE TO REPERTORY GRIDS
Following step 2 of the procedure, the sums of differences are shown below each construct, on the left. The reversed sums of differences are shown in bold on the right; you only need to reverse one set of ratings, for the ‘overall’ construct, in order to calculate them. The reversed ratings are shown in bold at the bottom. For each construct, the lower of the two values (unreversed, reversed) has been chosen and circled.
Following step 3, these sums of differences have been turned into % similarity scores.
Following step 4, the constructs have been divided into three sets, high, intermediate, and low, as evenly as possible.
Following step 5, the constructs have been labelled (2.1, 2.2, 2.3, etc.) to ensure subsequent identification. The grid sheet can now be cut up into strips, ready for categorisation.
Figure 7.1 Using Honey’s technique
(3)Ensure comparability with other grids. In other words, turn these sums of differences into % similarity scores.
(4)Take the individual’s personal metric into account. Look at these % similarity scores. Within each grid, divide the constructs as best you can into the highest third, intermediate third, and lowest third. (‘As best you can’. In

ANALYSING MORE THAN ONE GRID 175
Figure 7.1, 67%, 33% reversed, 67% reversed, 75%, and 65% don’t divide into three equal groups!)
(5)Label each construct with both indices. At this stage, as with the bootstrapping technique, it’s convenient if you transfer each construct onto a separate file card, and note which interviewee it came from. Or simply cut the grid sheet into strips, each construct on a separate strip; but be sure to indicate which interviewee’s construct it is! Give each construct its unique number, as suggested in Section 7.2.1 (construct 16.4 would be the fourth construct from interviewee number 16, for example). Now check that the % similarity scores have been written in below each construct. Next, mark it H, I, or L depending on its % similarity score value in comparison with the other constructs which that particular interviewee used. Now, back to the content-analysis procedure.
(6)Identify the categories.
(7)Allocate the constructs to the categories, following the core-categorisation procedure (see Section 7.2.1).
(8)Tabulate the result.
(9)Establish the reliability of the category system, following exactly the same procedures you used in steps 4.1 to 4.7 in Section 7.2.1. You get your colleague’s help in going through steps 6, 7, and 8, working with the constructs after they’ve been labelled with their % similarity scores and their H-I-L indices, but before doing anything else. There’s no point in summarising the table and doing differential analyses if the category system isn’t reliable.
(10)Summarise the table: first, the meaning of the category headings; that is, define the category headings.
(11)Summarise the table: find examples of each category heading. Here’s where the power of Honey’s approach reveals itself.
(11.1) Within each category, order the constructs from top to bottom with respect to their % similarity scores. Those at the top have identical or nearidentical scores (that is, they represent ‘what the interviewee particularly had in mind in thinking about the topic’, even though, within their category, of course, they may be different in meaning, covering some different aspect of that category). Those at the bottom, those with the low % similarity scores, will be less salient (bulking less, as it were, in that particular interviewee’s thinking so far as the topic is concerned).
11.2) Looking at all the constructs within a category, identify personally salient constructs on which there is consensus in the group.
.If the H-I-L indices are high, the idea behind that particular construct is important for the people in your sample as individuals. And if many individuals in your sample have that construct, then certainly, hang on

176 THE EASY GUIDE TO REPERTORY GRIDS
to it, since it’s saying something about the thinking of your sample as a whole as well as each individual member!
.If the H-I-L indices are mixed, the idea behind that particular construct reveals no particular consensus. In the sample as a whole, there’s a certain ambivalence about the construct’s relevance or importance to the topic. And particularly, if you notice that two or more constructs which express essentially the same meaning, obtained from the same person, have mixed H-I-L indices, set the constructs aside. There’s no point in preserving ambiguity or ambivalence here.
.If the H-I-L indices are low, it looks as though the sample as a whole agree that the construct doesn’t relate particularly well to the topic in general. Note this.
Table 7.9 shows the result of this step for our running example.
(11.3) If there are subthemes within a category, group them according to the meaning being expressed. The final result will reflect your overall purposes in doing a content analysis; but, typically, you would aim to end up with 40% to 80% of the original number of constructs which show the consensus you’re looking for. (Of course, if there was little consensus, you’d say so!) The result will be a table in which the columns are categories, each one divided into two subcolumns, in the first of which the chosen constructs appear, and, in the other, the % similarity scores and the H-I-L index.
(12) Summarise the table: state the frequency under the category headings. How many constructs are there in each category (and subcategory, if relevant) at this stage? Report the number, and if this varies markedly from the original number of constructs in each category at the start of step 10, assess and discuss the significance. On what kinds of issues is there a consensus, and on which ones don’t people agree?
This is a good point at which to calculate any sums, averages, and so on. You might, for example, use the % similarity scores to provide a ‘mean importance score’ of each category for the sample as a whole, if this makes sense in your analysis.
(13) Complete any differential analysis which your investigation requires, as before, when you bootstrapped. If you tag each construct according to the different subsamples of interviewee, or cross-tabulate them in different rows of your table, you may see differences among the subsamples in terms of:
. the number of constructs in different categories
.the relative importance (the H-I-L indices and/or the mean % similarity scores).

ANALYSING MORE THAN ONE GRID 177
So, for instance, suppose in our running example, you knew that interviewees nos 5, 6, and 7 had only had sales experience, whereas interviewees nos 1, 2, 3, and 4 had worked in the office before joining the sales force. Would the fact that there are more constructs from interviewees 5, 6, and 7 in the ‘pricing decisions’ category be meaningful?
But be careful! This example has only 50 interviewees since it is invented for illustrative purposes. A real data set would have at least 200 items. In point of fact, in this example, it would be dangerous to come to any differential conclusions because the subsamples of sales staff with office experience, and without, are rather small. For instance, four of the 10 constructs under the ‘pricing decisions’ category which we are using to make a point in our differential analysis come from just one interviewee, no. 7. We have to avoid a conclusion that the bee in one interviewee’s bonnet does indeed typify the views of the other interviewees in the subgroup!
As with any form of sampling, idiosyncrasies that do not reflect population characteristics are more probable in a small sample than a large one. If you want to conduct a differential analysis, make sure that you have sufficient constructs to represent each subgroup.
Okay. And, while on this statistical theme, note the following step.
(14) Complete any statistical tests on this differential analysis, as before.
Before moving on, make an attempt at Exercise 7.3. This gets you to practise steps 1 to 8 of the Honey procedure.
7.4 IN CONCLUSION
Content-analysis procedure, and particularly, the reliability check, are very time-consuming. Do we really have to follow them?
Well, what can I say? If accuracy and reliability matter to you, then the sane answer has to be ‘yes’, especially if, in the case of a dissertation based on repertory grid technique, your work is to be judged against scholarly standards by other people. And if accuracy and reliability don’t matter to you, should you really be using repertory grid technique in understanding other people?
Finally, I should mention that I have not described one very interesting bootstrapped content-analysis technique that aggregates individual interviewees’ grids while capturing information about the structure of their

Table 7.9 Content-analysis procedure: factors related to book sales as seen by publisher’s sales staff
|
|
|
No., |
% |
H-I-L |
Category |
|
Constructs |
% |
Similarity |
value |
|
|
|
|
|
|
Pricing decisions |
1.2 Affordable – less affordable at that size |
|
92 |
H |
|
|
3.1 |
Easily produced – difficult to produce at a reasonable price |
|
83 |
H |
|
4.4 |
For the under-£20 market – will sell at £25 |
|
100 |
H |
|
5.5 |
Easy to price – standard of illustration may make it too expensive |
10, |
83 |
I |
|
6.2 |
Looks like good value – looks too cheap to be worth the price |
20 |
75 |
I |
|
6.3 |
Under £20 and soft cover only – would need to sell for £35 |
|
92 |
H |
|
7.1 |
Cheap and cheerful – only libraries could afford it |
|
100 |
H |
|
7.2 |
Well advertised, could sell at that price – can’t produce it at the price |
|
92 |
H |
|
|
that would reach the buyer |
|
|
|
|
7.3 |
Affordable illustrative materials – requires a level of illustration we |
|
100 |
H |
|
|
couldn’t provide at the price |
|
|
|
|
7.7 |
Would sell at a higher price than normal for its market – would its |
|
58 |
L |
|
|
market buy something at the low price required? |
|
|
|
|
|
|
|
|
|
Buyer |
1.1 Well suited to the younger market – not our sort of customer |
|
100 |
H |
|
characteristics |
1.6 Appeal to intelligent lay reader – appeal to specialists |
|
75 |
I |
|
|
2.5 |
Pitched just right at readers – presentation too ‘‘pop’’ |
|
67 |
I |
|
3.7 |
A healthy undergraduate market – more postgraduate |
9, |
83 |
H |
|
4.6 |
Attractive, our sort of market – aimed at a more conservative sort of |
18 |
92 |
H |
|
|
customer? |
|
|
|
|
5.7 |
A good standard coverage – too narrowly focused for our market |
|
83 |
I |
|
6.1 |
Conventional cover, buyers will accept – ring-bound covers: buyers |
|
50 |
L |
|
|
don’t like |
|
|
|
|
6.4 Deals with the topics our market likes – some expected topics missing |
|
92 |
H |
|
|
6.6 |
Our sort of book – not sure if it’s for our readers |
|
58 |
L |
|
|
|
|
|
|
Competitors |
1.3 No current authors competing – several other competitors |
|
90 |
H |
|
|
2.1 |
Will take 2 years for this edition to be superseded – not aware of |
|
67 |
I |
|
|
immediate competitors’ plans |
|
|
|
|
2.4 |
No similar volume currently in print – several current competitors |
|
75 |
H |