
Solid-Phase Synthesis and Combinatorial Technologies
.pdf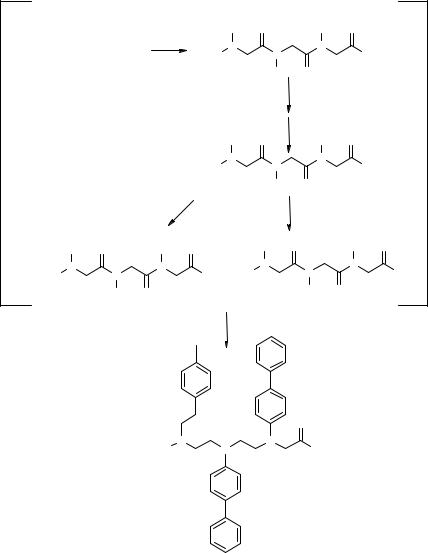

194 SYNTHETIC ORGANIC LIBRARIES: LIBRARY DESIGN AND PROPERTIES
|
|
R1 O |
R3 O |
|
|
|
|
|
N |
N |
|
|
|
|
H |
N |
NH2 |
|
|
|
|
|
R2 |
O |
|
|
|
|
|
R1-3 = 25 different alkyls or aryls |
|
|
|
|
|
15,625 virtual library components |
|
|
|
||
|
OH |
Me |
S |
HO |
|
|
|
|
|
|
|
|
|
|
O |
O |
|
O |
|
|
|
H |
H |
OH |
|
|
|
|
N |
N |
|
|
|
|
H2N |
N |
|
N |
|
H |
N |
|
H |
|
H |
HO |
Me |
|
|
O |
O |
O |
|
||
|
|
|
|
|||
|
5.4 |
|
|
H |
5.5 |
|
SELECTION using 5.4 as a LEAD: RECURRENT FRAGMENTS (10)
O |
OH |
COOH |
|
C |
I |
G |
|
Me |
|
A D
OMe |
H |
OMe |
|
J |
|||
E |
|
||
N O |
OMe |
||
|
|
O |
NH2 |
|
B |
O |
|
F |
||
|
SELECTION using 5.5 as a LEAD: RECURRENT FRAGMENTS (5)
O
B G I
O
K L
Figure 5.24 Virtual screening of a 15,625-membered tripeptoid virtual library using SA-based selection: met-enkephalin 5.4 and morphine 5.5 as lead compounds for computational selection.
binding activity, and for each performed four series of 40 SA similarity-based selection cycles on the same 15,625-membered virtual tripeptoid library. The results of these series, expressed as the occurrence of each building block in the 50 most similar structures found, are reported for both lead structures in Fig. 5.24. The two nonpeptoid leads produced complementary patterns, and the selection of the 12 building blocks A–L with a statistically significant occurrence would have found all the three active tripeptoids (Fig. 5.25) out of the original library tested for -opiate activity (109). A

|
|
|
|
|
|
|
|
|
|
|
5.4 LIBRARY DESIGN VIA COMPUTATIONAL TOOLS |
195 |
|||||||||||||||||||||
A |
O |
|
|
I |
O |
|
|
|
|
|
|
|
|
|
A |
O |
|
|
I |
O |
|||||||||||||
|
|
|
|
|
|
|
|
|
|
|
|
|
|
|
|
|
|
|
|
|
|
|
|
|
|
|
|
|
|
|
|
|
|
N |
|
|
N |
|
|
N |
|
|
NH2 |
|
|
|
|
|
|
|
|
|
N |
|
|
|
|
|
N |
|
|
|
|||||
H |
|
|
|
|
|
|
|
|
|
|
|
|
|
|
|
|
|
|
|
N |
|
|
|
|
NH |
||||||||
|
|
|
|
|
|
|
|
|
|
|
|
|
|
|
|
|
H |
|
|
|
|
|
|
|
|
||||||||
|
|
|
|
L |
|
|
|
|
|
|
|
|
|
|
|
|
|
|
|
|
|
|
|
|
|
|
|
|
|
|
2 |
||
|
|
|
|
O |
|
|
A |
O |
|
|
I |
O |
|
|
J |
O |
|
|
|
||||||||||||||
|
|
5.6 |
|
|
|
|
|
|
|
|
|
|
|
|
|
||||||||||||||||||
|
|
|
|
|
|
|
|
|
|
|
|
|
|
|
|
|
|
|
|
|
|
|
|
5.8 |
|
|
|
|
|
|
|||
|
|
|
|
6 nM |
|
|
|
|
|
|
N |
|
|
N |
|
|
N |
|
|
NH2 |
|
|
|
|
|
|
|
|
|
||||
|
|
|
|
|
|
|
|
|
|
H |
|
|
|
|
|
|
|
|
|
|
31 nM |
|
|
|
|||||||||
|
|
|
|
|
|
|
|
|
|
|
|
|
|
|
|
|
|
|
|
|
|||||||||||||
|
|
|
|
-opiate |
|
|
|
|
|
|
D |
|
|
|
|
|
|
|
|
|
|
-opiate |
|
|
|
||||||||
|
|
|
|
|
|
|
|
|
|
O |
|
|
|
|
|
|
|
|
|
||||||||||||||
|
|
|
|
|
|
|
|
|
|
|
|
|
|
|
|
|
|
|
|
|
|
|
|
5.7
46 nM-opiate
SELECTION using 5.4 (met-enkephalin) as a LEAD structure: A, D, I, J (4 out of 5) detected
SELECTION using 5.5 (morphine) as a LEAD structure: I, L (2 out of 5) detected
SELECTION using 5.4 and 5.5 as LEAD STRUCTURES: 12 substituents detected, 5 out of 5 from-opiate positives
Figure 5.25 Virtual screening of a 15,625-membered tripeptoid virtual library using SA-based selection: final outcome leading to nonpeptoidic leads.
library of 123 = 1728 compounds would have thus been sufficient to cover the significant activity/similarity profile embedded in the whole virtual library (253 = 15,625 virtual components). Another report highlighting the potential of simulated annealing guided evaluation (SAGE) in library design has recently been published (110).
Two other examples of rational design of focused libraries are related to the exploitation of target information, and use either Genetic Optimization for Ligand Docking (GOLD) (111–113) to screen virtual combinatorial libraries for their docking with a partially flexible target using a genetic algorithm; or the ligand design program LUDI (114, 115) to map the active site of a target and to extract the structural information needed to build a target-focused small library.
Jones et al. (113) selected as target a lipase from Candida rugosa with known active site coordinates (116) and built a 44,730-member virtual library of amides L1 from commercially available acids (M1, 426 candidates) and amines (M2, 105 candidates) (Fig. 5.26). The selection was performed on reagents, as the library size would have prevented the docking of each reaction product; each monomer, though, was “adjusted” to resemble the final amides by converting acids M1 to N-methylamides M1R and amines M2 to acetylamino compounds M2R (a, Fig. 5.26). Each adjusted monomer was docked into the lipase active site using GA to select the ideal GOLD fit; three 50,000 operation-GA runs per monomer were performed to select three different fits, unless the first two results were already extremely similar (b, Fig. 5.26). A total of 1076 GOLD fits for the acids and 270 fits for amines were identified. The fits were then merged to build the more meaningful amides from the virtual library: merging was done deleting the overlapping parts of M1R and M2R and joining the remaining fragments, providing that they were in the correct orientation to be linked and that the resulting amides did not clash with the active site structure. Only 311 combinations

5.4 LIBRARY DESIGN VIA COMPUTATIONAL TOOLS 197
number of actual versus virtual monomers (>10 for acids, >2 for amines); a confirmation of the virtual screening results via resynthesis and testing of the selected individuals would be crucial to validate the process. The authors cross-checked computationally the results by docking the 129 selected compounds into the lipase active site using a more rigorous GA-selection procedure to find the preferred docking conformations (10 GA runs per compound, 100,000 operations per run); the vast majority (>85%) of compounds gave a good correlation between reagent-based and product-based binding mode.
Lew and Chamberlin (115) selected the human T cell Kv1.3 potassium channel as a target for novel immunosuppressive agents (117). Using LUDI (114, 115) the target active site was screened by docking into it over 1,000 random structural fragments (e.g., amines, acids, phenyl rings); the essential active site residues for specific Kv1.3 blockade and the optimal location of hydrophobic, hydrophilic and hydrogen bond donor/acceptor groups in the site were determined (see ref. 115 for more details). The specificity of the built model was successfully validated by docking known, selective Kv1.3 blockers (complete match with the LUDI model) and aspecific blockers (partial mismatch). The identified fragments were then connected respecting their orientation and distances; this resulted in the selection of a phenyl stilbene scaffold (5.9, Fig. 5.27) as a compromise between ideal docking, restricted conformational freedom and reasonable binding energies. The general fit of the scaffold to the target active site was exploited by combinatorial modification of three randomization points (R1–R3), and a synthetic scheme for a scaffold 5.9-derived library employing commercially available reagents and monomers was designed and assessed (Fig. 5.27). A 400-member discrete library L3 was prepared (Fig. 5.28) and screened discovering several weakly active channel blockers (e.g., 5.10–5.13, Fig. 5.28); a preliminary, but assessed SAR for the phenyl stilbene scaffold which could be used for further optimizations was also obtained.
Computational design/selection tools for focused libraries can significantly reduce the efforts required to optimize preliminary structural information while ensuring the exploitation of the same structural input. The use of these results to refine the structural requirements and to eventually generate better focused libraries will become a key tool for combinatorial scientists working with synthetic organic libraries for different applications.
5.4.3 Biased-Targeted Libraries
Concurrent to the increasingly popular use of diverse libraries to sample chemical space and to provide chemical leads for various applications, the search for areas inside chemical space that are more promising for specific applications has also gained relevance (118–120). We will review the example of pharmaceutical research, where the so-called druglike properties of a molecule are the subject of frequent reports by various groups.
It has been observed that most of the currently available drugs are small organic molecules, and their molecular weight is typically between 250 and 600 daltons. With this constraint introduced for library design, and knowing that at least two monomer

198 SYNTHETIC ORGANIC LIBRARIES: LIBRARY DESIGN AND PROPERTIES
R1
5.9
|
R2 |
|
R3 |
|
|
|
|
O |
|
|
|
|
|
N |
|
|
|
|
|
|
H |
|
|
|
O |
|
O |
|
|
|
+ |
|
|
R1 |
|
SC |
M1 |
|
|
||
PPh3 |
|
|
|
||
N |
|
|
|
|
|
H |
|
|
|
|
|
|
Br |
|
Br |
|
|
|
|
|
|
|
|
|
O |
R1 |
|
|
O |
|
|
|
|
R1 |
|
M2 |
|
|
SC |
M3 |
R3 N |
|
|
|
|
|
H |
|
|
activation |
|
5.9 |
|
R2 |
|
|
|
|
|
|
|
|
|
R2 |
SC = safety-catch linker
M1 = aromatic aldehydes
M2 = aromatic boronic acids
M3 = nucleophiles
Figure 5.27 Design of an SP route to a focused SP library of phenyl stilbenes selected with the program LUDI for the Kv1.3 potassium channel.
sets are to be used for a library synthesis, each monomer must weigh 250 daltons or even less, thus strongly limiting the number of candidate monomers for each chemical class. The lipophilicity of organic molecules is also extremely important to determine their usefulness as drugs. Typically, this property, expressed as the partition coefficient of a molecule between n-octanol and water (log P), is ideal for drug discovery when it is between the logarithmic values of 2 and 4–5, handicapping both extremely hydrophilic and lipophilic building blocks. Another popular filter is the number of rotatable bonds contained in a library individual: When compounds are extremely flexible, their binding capacity, related to a specific conformation, becomes weaker so that significant libraries should always contain a reasonable degree of rigidity (possibly embedded into the scaffold, but also present from the monomer) to act effectively as a source of relevant hits. Computational chemistry may help in both evaluating the degree of flexibility of molecules and subsets and selecting compounds having predefined profiles in terms of degrees of freedom. These and other properties can

5.4 |
LIBRARY DESIGN VIA COMPUTATIONAL TOOLS 199 |
|
R1 |
|
L3 |
R2 |
400-member |
SP discrete library |
|
|
R3 |
|
O N |
H
R1 include unsubstituted; 2-F; 4-F; 3-F,4-Cl; 3,4-diOBn; 3-Cl; 4-OBn
R2 include unsubstituted; 4-Cl; 4-OMe; 4-F
R3 include Me; Et; n-Pr; i-Pr; n-Bu; cyclohexyl; guanidyl; morpholyl
SELECTED CHANNEL BLOCKERS:
|
|
|
|
|
OBn |
|
Cl |
|
|
|
|
|
OBn |
|
F |
Cl |
|
O |
Me |
|
Cl |
O |
N |
|
N |
|
|||||
|
|
|
H |
|
|
|
H |
IC |
|
= 2.9 M |
5.10 |
|
IC50 = 3.7 M |
5.11 |
|
50 |
|
|
|||||
|
|
|
|
|
F |
|
|
|
|
|
|
|
|
|
F |
|
|
|
H |
NH |
|
|
|
|
|
|
N |
Cl |
O |
N |
|
Cl |
|
O |
N |
|
|||
|
|
|
|
H |
|||
|
|
|
H |
NH2 |
|
IC50 = 8.0 M |
5.13 |
|
IC50 = 3.9 M |
|
|
||||
|
|
|
|
|
|
5.12
Figure 5.28 Structure of the phenyl stilbene focused library L3 and of several positives from screening (5.10–5.13).
easily be predicted by software programs, as shown in several papers (75, 121–123), to aid selection among the virtual sets comprised in the druglike chemical space.
A recent report presented the so-called MultiLevel Chemical Compatibility (MLCC) to determine the similarity of any structure to known drug-compatible compounds from representative databases (124); although by definition incomplete, as all the “drug-like” space is far from being determined, this and similar tools can


