
Advanced Wireless Networks - 4G Technologies
.pdf
6
Teletraffic Modeling
and Analysis
Traditional traffic models have been developed for wireline networks. These models predict the aggregate traffic going through telephone switches. Queueing theory is the tool which has been traditionally used in the analysis of such systems. A summary of the main results from the queueing theory is included in Appendix C (please go to www.wiley.com/go/glisic). These traditional models do not include subscriber mobility or callee distributions and therefore need modifications to be applicable for modeling the traffic in wireless networks.
6.1 CHANNEL HOLDING TIME IN PCS NETWORKS
Channel holding (occupancy) time is an important quantity in teletraffic analysis of PCS networks. It corresponds to service time in conventional queueing theory. This quantity is needed to derive key network design parameters such as the new call blocking probability and the handoff call blocking probability [1]. The cell residence time is a nonnegative random variable, so a good distribution model for the random variable will be sufficient for characterizing the users’ mobility. In this section we use, the hyper-Erlang distribution model [2] for such purposes.
The hyper-Erlang distribution has the following probability density function and Laplace transform:
M |
|
|
(mi ηi )mi tmi −1 |
|
||||
|
|
|
|
|||||
fhe(t) = i=1 |
αi |
|
|
|
|
|
e−mi ηi t , |
t ≥ 0 |
|
|
(mi − 1)! |
|
|||||
M |
|
|
|
mi ηi |
|
mi |
|
|
fhe*(s) = i=1 |
αi |
|
|
|
|
(6.1) |
||
|
|
s + mi ηi |
|
|
||||
|
|
|
|
|
|
|||
Advanced Wireless Networks: 4G Technologies |
|
Savo G. Glisic |
|
|
|
|||
C 2006 John Wiley & Sons, Ltd. |
|
|
|
|
|
|
|
|

180 |
TELETRAFFIC MODELING AND ANALYSIS |
|
|||
where αi ≥ 0, and |
M |
αi = 1. |
M, m1, m2, . . . , mM |
are nonnegative integers and η1, |
|
i=1 |
η2, . . . ,ηM are positive numbers. These distribution functions provide sufficiently general models, i.e. hyper-Erlang distributions are universal approximations.
It can be shown [3] that for a given cumulative distribution function G(t) of a nonnegative random variable we can choose a sequence of distribution functions Gm (t), each of which corresponds to a mixture of Erlang distributions, so that limm→∞ Gm (t) = G(t) for all t at
which G(t) is continuous. Gm (t) can be chosen as |
|
|
|
|
|
|
|||||||||
G |
|
(t) |
= |
∞ |
G |
k |
− |
G |
k − 1 |
Gk |
(t), |
t |
≥ |
0 |
(6.2) |
m |
|
m |
m |
||||||||||||
|
|
k=1 |
|
|
m |
|
|
|
|
||||||
|
|
|
|
|
|
|
|
|
|
|
|
|
|
|
where Gkm (t) is the distribution function of an Erlang distribution with mean k/m and variance k/m2 (the distribution of the sum of k exponential random variables each with mean 1/m). If gm (t) and gm*(s) are the density function and Laplace transform of Gm (t), and gmk (t) is the density function of Gkm (t) then we have
∞
gm (t) =
k=1
∞
gm*(s) =
k=1
G |
k |
− |
G |
k − 1 |
gk |
(t) |
|
|
||
m |
m |
|
|
|||||||
|
|
m |
|
|
|
|
||||
|
k |
|
|
k − 1 |
|
|
|
k/m |
k |
|
G |
− |
G |
|
|
|
(6.3) |
||||
m |
m |
|
s |
+ |
k/m |
|||||
|
|
|
|
|||||||
|
|
|
|
|
where the asterisk is used to denote the Laplace transformation.
The resulting distribution is called the ‘mixed Erlang distribution’. Their coefficients can be determined from the experimental data. If a finite number of terms is used to approximate the distribution function, the resulting distribution approximates the hyper-Erlang distribution.
To illustate why the distribution Gm (t) provides the universal approximation to general distribution models we show Erlang distribution
|
(mη)mtm−1 |
|
|
|||
fe(t) = |
|
|
|
e−mηt , |
t ≥ 0 |
(6.4) |
(m |
− |
1)! |
||||
|
|
|
|
|
|
in Figure 6.1 by varying the shape parameter m (Appendix C; please go to www.wiley
.com/go/glisic). We observe that, as the shape parameter m becomes sufficiently large, the density function approaches the Dirac δ function. Hence, fe(t) approaches the δ function as m is sufficiently large. From signal processing theory [4], we know that the δ function can be used to sample a function and reconstruct the function from the sampled data (the sampling theorem). We can replace the δ function by the Erlang density function with sufficiently large m, and the resulting approximation is exactly in the form of the hyper-Erlang distribution.
If the cell residence time t is modeled by the hyper-Erlang distribution as in Equation (6.1), its kth moment is given as
E tk |
|
|
|
1)k f *(k) |
|
M |
|
(mi + k − 1)! |
|
|
η )−k |
|
||
= |
( |
− |
(0) |
= i=1 |
α |
|
(m |
(6.5) |
||||||
(mi − 1)! |
||||||||||||||
|
|
|
he |
|
i |
i |
i |
|
||||||
The parameters αi , mi and ηi |
(i = 1, 2, . . . , M) can be found by fitting a number of mo- |
ments from field data. Moreover, if the number of moments exceeds the number of variables,

CHANNEL HOLDING TIME IN PCS NETWORKS |
181 |
|
2.4 |
|
|
|
|
|
|
|
|
|
2 |
|
|
|
|
|
|
|
|
|
|
|
|
m = 100 |
|
|
|
|
|
density |
1.6 |
|
|
|
|
|
|
|
|
|
|
|
m = 50 |
|
|
|
|
|
|
|
|
|
|
|
|
|
|
|
|
Probability |
1.2 |
|
|
|
|
|
|
|
|
0.8 |
|
m = 20 |
|
|
|
|
|
||
|
|
|
|
|
|
|
|||
|
|
|
|
|
|
|
|
||
|
|
m = 1 |
|
|
|
|
|
|
|
|
|
|
m =5 |
|
|
|
|
|
|
|
0.4 |
|
|
|
|
|
|
|
|
|
0 |
|
|
|
|
|
|
|
|
|
0 |
0.5 |
1 |
1.5 |
2 |
2.5 |
3 |
3.5 |
4 |
|
|
|
|
|
Time |
|
|
|
|
Figure 6.1 Probability density function for Erlang distribution.
|
|
|
|
tc |
|
|
|
||
|
|
|
|
|
|
|
|||
|
|
t1 |
|
|
|
rm |
|
|
|
|
|
|
|
|
|
||||
|
|
|
|
|
|
|
|
|
|
|
|
r1 |
t2 |
|
|
tm |
tm+1 |
|
|
|
|
|
|
|
|
|
|
|
|
|
|
|
|
|
|
|
|
|
|
Figure 6.2 The call holding and cell residence times.
then the least-square method can be used to find the best fit to minimize the least-square error.
The channel holding time distribution depends on the mobility of users, which can be characterized by the cell residence time [1–30, 5–31]. In the sequel we use the following notation: tc, call holding time (exponentially distributed with parameter μ); tm , cell residence time; r1, time between the instant a new call is initiated and the instant the new call moves out of the cell if the new call is not completed; rm (m > 1), residual life time distribution of call holding time when the call finishes mth handoff successfully; and tnh and thh, the channel holding times for a new call and a handoff call, respectively. Then, from Figure 6.2, the channel holding time for a new call will be
tnh = min {tc, r1} |
(6.6) |
and the channel holding time for a handoff call is
thh = min {rm , tm } |
(6.7) |
182 TELETRAFFIC MODELING AND ANALYSIS
Let tc, tm , r1, thh and tnh have density functions fc(t), f (t), fr(t), fhh(t) and fnh(t) with their corresponding Laplace transforms fc*(s), f *(s), fr*(s), fhh*(s) and fnh*(s), respectively, and with cumulative distribution functions, fc(t), F(t), Fr (t), Fhh(t) and Fnh(t) respectively. From Equation (6.7) we obtain the probability
Pr(thh ≤ t) = Pr (rm ≤ t or tm ≤ t) |
|
= Pr (rm ≤ t) + Pr (tm ≤ t) − Pr (rm ≤ t, tm ≤ t) |
|
= Pr (rm ≤ t) + Pr (tm ≤ t) − Pr (rm ≤ t) Pr (tm ≤ t) |
(6.8) |
= Pr (tc ≤ t) + Pr (tm ≤ t) − Pr (tc ≤ t) Pr (tm ≤ t) |
|
which is based on the independency of rm and tm , and the memoryless property of the exponential distribution from which we have that the distribution of rm has the same distribution as tc. Differentiating Equation (6.8), gives
|
|
|
|
|
|
fhh(t) |
|
= fc(t) + f (t) − fc(t) Pr(tm ≤ t) − Pr(tc ≤ t) f (t) |
|
|||||||||||||||||||
|
|
|
|
|
|
|
|
|
|
|
= fc (t) |
|
∞ |
|
|
|
∞ |
|
|
|
|
|
|
|
||||
|
|
|
|
|
|
|
|
|
|
|
t |
f (τ ) dτ + f (t) |
t |
fc(τ ) dτ |
|
|
|
(6.9) |
||||||||||
and |
|
|
|
|
|
|
|
|
|
|
|
|
|
|
|
|
|
|
|
|
|
|
|
|
|
|
|
|
|
* |
|
|
f *(s) |
|
f * |
|
|
|
|
|
∞ |
|
|
t |
|
|
|
|
∞ |
|
|
|
t |
||||
f |
(s) |
= |
+ |
(s) |
− |
|
|
e−st f (t) |
|
f |
(τ ) dτ dt |
− |
e−st f |
(t) |
f (τ ) dτ dt |
|||||||||||||
hh |
|
|
|
|
|
c |
|
|
|
0 |
|
∞ |
o |
c |
|
|
0 |
c |
|
|
o |
|||||||
|
* |
|
|
f *(s) |
|
f * |
|
|
|
|
|
|
|
t |
|
|
|
|
∞ |
|
|
|
|
|||||
f |
(s) |
= |
+ |
(s) |
− |
μ |
|
e−(s+μ)t |
|
f (τ ) dτ dt |
− |
|
e−st (1 |
− |
e−μt ) f (t) dt |
|||||||||||||
hh |
|
|
|
|
|
c |
|
s |
|
|
0 |
|
|
o |
|
|
|
0 |
|
|
|
|||||||
|
|
|
= |
|
μ |
|
+ |
|
|
|
|
f |
*(s + μ) |
|
|
|
|
|
|
|
|
|
|
(6.10) |
||||
|
|
|
|
|
|
|
|
|
|
|
|
|
|
|
|
|
|
|
|
|||||||||
|
|
|
s |
+ |
μ |
s |
+ |
μ |
|
|
|
|
|
|
|
|
|
|
||||||||||
|
|
|
|
|
|
|
|
|
|
|
|
|
|
|
|
|
|
|
|
|
|
|
|
|
|
From the above equations, we obtain the expected handoff call channel holding time as
|
1 |
|
|
|
|
E [thh] = − fhh* (0) = |
|
|
[1 |
− f *(μ)] |
(6.11) |
μ |
where fhh* is the first derivative of the function. Starting from Equation (6.6) and using a similar argument, we obtain
|
|
|
|
|
∞ |
|
|
|
|
|
∞ |
|
fnh(t) = fc(t) |
t |
|
fr(τ ) dτ + fr(t) |
t |
fc(τ ) dτ |
|||||||
fnh*(s) = |
|
μ |
|
|
+ |
|
s |
|
|
fr*(s + μ) |
|
(6.12) |
|
|
|
|
|
|
|
|
|
||||
s |
+ |
μ |
|
s |
+ |
μ |
|
|||||
|
|
|
|
|
|
1 |
|
|
|
|
||
|
|
*(1) |
|
|
|
|
* |
|
|
|||
E [tnh] = − fnh |
|
(0) = |
|
|
1 − fr (μ) . |
|||||||
|
μ |
We need to consider the channel holding time distribution for any call (either new call or handoff call), i.e. the channel holding time for the merged traffic of new calls and handoff calls. We will simply call this the channel holding time, using no modifiers such as new call or handoff call. If th is the channel holding time and λh the handoff call arrival rate, and λ is the new call arrival rate then, th = tnh with probability λ(λ + λh) and th = thh with probability λh(λ + λh). Let fh(t) and fh*(s) be its density function and the corresponding

CHANNEL HOLDING TIME IN PCS NETWORKS
Laplace transform. It is easy to obtain |
|
|
|
|
|||
fh(t) = |
λ |
fnh |
(t) + |
λh |
fhh(t) |
|
|
|
|
|
|||||
λ + λh |
λ + λh |
|
|||||
fh*(s) = |
λ |
fnh* |
(s) + |
λh |
fhh*(s) |
|
|
λ + λh |
λ + λh |
|
|||||
E [th] = |
λ |
|
1 − fr*(μ) + |
λh |
1 − f *(μ) |
||
μ(λ + λh) |
μλ + λh) |
183
(6.13)
When the residual lifetime r1 of t1 is exponentially distributed with parameter μr , then its Laplace transform fr*(s) is μr/(s + μr). Using this in Equation (6.12), results in
f * |
(s) |
|
μ |
|
|
|
μrs |
|
μ + μr |
(6.14) |
|
= s + μ |
+ |
(s + μ)(s + μ + μr) |
= s + μ + μr |
||||||||
nh |
|
|
which implies that the new call channel holding time is exponentially distributed with parameter μ + μr. Similarly, if the cell residence time ti is exponentially distributed with parameter η, then the handoff call channel holding time is also exponentially distributed with parameter μ + η. In this case, the channel holding time is hyperexponentially distributed. If μr = η, then the channel holding time [see Equation (6.12)] is exponentially distributed with parameter μ + η. In fact, since r1 is the residual life of t1, from the Residual Life Theorem [21], we have
* |
(s) = |
η 1 − f *(s) |
|
|
η |
|
= f |
* |
(s) |
(6.15) |
fr |
s |
= s |
+ |
η |
|
|||||
|
|
|
|
|
|
|
|
|
|
Hence, the channel holding time is exponentially distributed with parameter μ + η when the cell residence time is exponentially distributed.
Simple results for the conditional distribution for channel holding time when the cell residence time is generally distributed are presented next. Let fcnh(t), fchh(t) and fch(t) denote the conditional density functions for new call channel holding time, the handoff call channel holding time and the channel holding time, respectively, with Laplace transforms
fcnh* (s), fchh* (s) and fch*(s), and with cumulative distribution functions and Fcnh(t), Fchh(t) and Fch(t). Let us start with the conditional distribution for the handoff call channel holding
time. We have
|
|
|
|
|
|
|
|
|
|
|
|
|
|
|
|
|
|
|
Pr(rm ≤ h, rm ≤ tm ) |
|
|
|
h |
f |
(t) |
∞ f (τ ) dτ dt |
||||||
F |
(h) |
= |
Pr(t |
hh ≤ |
h |
| |
r |
|
|
≤ |
t |
|
) |
= |
|
= |
|
0 |
c |
|
t |
|
||||||||||
|
|
|
|
|
|
|
Pr(rm ≤ tm ) |
|||||||||||||||||||||||||
chh |
|
|
0h |
|
|
m |
|
|
m |
|
|
Pr(rm ≤ tm ) |
|
|
|
|
|
|||||||||||||||
|
|
= |
|
fc(t) [1 − F(t)] dt |
|
|
|
|
|
|
|
|
|
|
|
|
|
(6.16) |
||||||||||||||
|
|
|
|
Pr(rm ≤ tm ) |
|
|
|
|
|
|
|
|
|
|
|
|
|
|
|
|
|
|
|
|||||||||
Differentiation of both sides gives the conditional density function |
|
|
|
|||||||||||||||||||||||||||||
|
|
|
|
|
|
|
|
|
|
|
f |
chh |
(h) |
= |
fc(h) [1 − F(h)] |
|
|
|
|
|
|
(6.17) |
||||||||||
with |
|
|
|
|
|
|
|
|
|
|
|
|
|
|
|
Pr(rm ≤ tm ) |
|
|
|
|
|
|
|
|
|
|||||||
|
|
|
|
|
|
|
|
|
|
|
|
|
|
|
|
|
|
|
|
|
|
|
|
|
|
|
|
|
|
|
|
|
|
|
Pr(rm ≤ tm ) = |
|
∞ |
|
|
t |
f (t) fc(τ ) dτ dt = |
∞ |
f (t) |
1 − eμt dt |
|||||||||||||||||||||
|
|
|
0 |
|
|
|
0 |
|
|
0 |
|
|||||||||||||||||||||
|
|
|
|
|
|
|
= |
1 |
− |
|
|
|
|
∞ |
f (t)e−μt dt |
= |
1 |
− |
f *(μ) |
|
|
|
(6.18) |
|||||||||
|
|
|
|
|
|
|
|
|
|
|
|
|
|
|
|
|||||||||||||||||
|
|
|
|
|
|
|
|
|
|
|
|
|
|
|
|
|
|
|
|
|
|
|
|
|
|
|
0
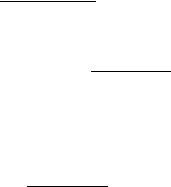

|
|
|
|
|
|
|
|
CHANNEL HOLDING TIME IN PCS NETWORKS |
185 |
|||
gives |
|
|
|
|
|
|
|
|
|
|
|
|
|
|
|
|
(1 |
|
|
|
|
|
fr*(s) |
* |
|
E |
[ |
H |
] = − |
− |
p |
bn) |
Res |
|
|
fc (−s) |
(6.24) |
|
|
− (1 − pbh) f *(s) |
|||||||||||
|
|
|
|
P σc s= p s 1 |
|
where σc denotes the set of poles of fc*(−s) in the right half of the complex plane and Ress= p denotes the residue at the pole s = p. Since tc is exponentially distributed with parameter μ, hence fc*(s) = μ/(s + μ), from the above we obtain
E [H ] |
= |
(1 − pbn) fr*(μ) |
(6.25) |
|
1 − (1 − pbh) f *(μ) |
||||
|
|
Since each unblocked call initiates E[H ] handoff calls on average, the handoff call arrival
rate can be obtained: |
|
|
|
|
|
|
|
λ |
h = |
λE [H ] |
= |
|
(1 − pbn)λ fr*(μ) |
(6.26) |
|
1 − (1 − pbh) f *(μ) |
|||||||
|
|
|
As long as fr*(s) and f *(s) are proper rational functions, then the Laplace transforms of distribution functions of all channel holding times (either conditional or unconditional) are all rational functions. To find the corresponding density functions, we only need to find the inverse Laplace transforms. This can be accomplished by using the partial fractional expansion [32].
As an illustration, suppose that g(s) is a proper rational function with poles p1, p2, . . . , pk with multiplicities n1, n2, . . . , nk . Then g(s) can be expanded as
|
|
|
|
k |
ni |
|
|
|
s j |
|
|
|
|
|||
|
|
|
g(s) = i=1 j=0 Ai j |
|
|
|
|
|
|
|
|
|
|
|||
|
|
(s + pi )ni |
|
|
|
|
||||||||||
where the constants can be found easily by the formula |
|
|
|
|
||||||||||||
Ai j = |
d j |
(s + pi )ni g(s) s=0 , j = 0, 1, . . . , i, |
i = 1, 2, . . . , k |
|||||||||||||
ds j |
||||||||||||||||
The inverse Laplace transform L−1 gives |
|
|
|
|
|
|
|
|
|
|
|
|
||||
|
|
|
d j |
|
|
|
|
|
|
|
|
|
|
th |
||
L−1 s j |
f (s) = |
|
L−1 [ f (s)] |
, L−1 |
1/(s + |
β)h |
= |
|
e−βt |
|||||||
dt j |
h! |
|||||||||||||||
and |
|
|
|
|
|
|
|
|
|
|
|
|
|
|
|
|
|
|
|
|
k |
mi |
|
|
d j |
|
t j |
|
|
|
|
||
|
|
L−1 [g(s)] = |
|
Ai j |
|
|
|
e− pi t |
|
|
|
|||||
|
|
j=0 |
dt j |
j! |
|
|
|
|||||||||
|
|
|
|
i=1 |
|
|
|
|
|
|
|
|
|
|
|
At this stage it is useful to remember that the inverse Laplace transform of a rational function is in fact the impulse response of a linear system in which the rational function is the system transfer function of the resulting linear system [32] and the cumulative distribution function is the step response of the linear system. In Matlab, the commands impulse and step can be used to find the density function and the distribution function. When applying the hyper-Erlang distribution model for cell residence time, we can in fact reduce the computation further. Substituting f *(s) in Equation (6.10) with fhe*(s) given by Equation (6.1),

