
- •Biological and Medical Physics, Biomedical Engineering
- •Medical Image Processing
- •Preface
- •Contents
- •Contributors
- •1.1 Medical Image Processing
- •1.2 Techniques
- •1.3 Applications
- •1.4 The Contribution of This Book
- •References
- •2.1 Introduction
- •2.2 MATLAB and DIPimage
- •2.2.1 The Basics
- •2.2.2 Interactive Examination of an Image
- •2.2.3 Filtering and Measuring
- •2.2.4 Scripting
- •2.3 Cervical Cancer and the Pap Smear
- •2.4 An Interactive, Partial History of Automated Cervical Cytology
- •2.5 The Future of Automated Cytology
- •2.6 Conclusions
- •References
- •3.1 The Need for Seed-Driven Segmentation
- •3.1.1 Image Analysis and Computer Vision
- •3.1.2 Objects Are Semantically Consistent
- •3.1.3 A Separation of Powers
- •3.1.4 Desirable Properties of Seeded Segmentation Methods
- •3.2 A Review of Segmentation Techniques
- •3.2.1 Pixel Selection
- •3.2.2 Contour Tracking
- •3.2.3 Statistical Methods
- •3.2.4 Continuous Optimization Methods
- •3.2.4.1 Active Contours
- •3.2.4.2 Level Sets
- •3.2.4.3 Geodesic Active Contours
- •3.2.5 Graph-Based Methods
- •3.2.5.1 Graph Cuts
- •3.2.5.2 Random Walkers
- •3.2.5.3 Watershed
- •3.2.6 Generic Models for Segmentation
- •3.2.6.1 Continuous Models
- •3.2.6.2 Hierarchical Models
- •3.2.6.3 Combinations
- •3.3 A Unifying Framework for Discrete Seeded Segmentation
- •3.3.1 Discrete Optimization
- •3.3.2 A Unifying Framework
- •3.3.3 Power Watershed
- •3.4 Globally Optimum Continuous Segmentation Methods
- •3.4.1 Dealing with Noise and Artifacts
- •3.4.2 Globally Optimal Geodesic Active Contour
- •3.4.3 Maximal Continuous Flows and Total Variation
- •3.5 Comparison and Discussion
- •3.6 Conclusion and Future Work
- •References
- •4.1 Introduction
- •4.2 Deformable Models
- •4.2.1 Point-Based Snake
- •4.2.1.1 User Constraint Energy
- •4.2.1.2 Snake Optimization Method
- •4.2.2 Parametric Deformable Models
- •4.2.3 Geometric Deformable Models (Active Contours)
- •4.2.3.1 Curve Evolution
- •4.2.3.2 Level Set Concept
- •4.2.3.3 Geodesic Active Contour
- •4.2.3.4 Chan–Vese Deformable Model
- •4.3 Comparison of Deformable Models
- •4.4 Applications
- •4.4.1 Bone Surface Extraction from Ultrasound
- •4.4.2 Spinal Cord Segmentation
- •4.4.2.1 Spinal Cord Measurements
- •4.4.2.2 Segmentation Using Geodesic Active Contour
- •4.5 Conclusion
- •References
- •5.1 Introduction
- •5.2 Imaging Body Fat
- •5.3 Image Artifacts and Their Impact on Segmentation
- •5.3.1 Partial Volume Effect
- •5.3.2 Intensity Inhomogeneities
- •5.4 Overview of Segmentation Techniques Used to Isolate Fat
- •5.4.1 Thresholding
- •5.4.2 Selecting the Optimum Threshold
- •5.4.3 Gaussian Mixture Model
- •5.4.4 Region Growing
- •5.4.5 Adaptive Thresholding
- •5.4.6 Segmentation Using Overlapping Mosaics
- •5.6 Conclusions
- •References
- •6.1 Introduction
- •6.2 Clinical Context
- •6.3 Vessel Segmentation
- •6.3.1 Survey of Vessel Segmentation Methods
- •6.3.1.1 General Overview
- •6.3.1.2 Region-Growing Methods
- •6.3.1.3 Differential Analysis
- •6.3.1.4 Model-Based Filtering
- •6.3.1.5 Deformable Models
- •6.3.1.6 Statistical Approaches
- •6.3.1.7 Path Finding
- •6.3.1.8 Tracking Methods
- •6.3.1.9 Mathematical Morphology Methods
- •6.3.1.10 Hybrid Methods
- •6.4 Vessel Modeling
- •6.4.1 Motivation
- •6.4.1.1 Context
- •6.4.1.2 Usefulness
- •6.4.2 Deterministic Atlases
- •6.4.2.1 Pioneering Works
- •6.4.2.2 Graph-Based and Geometric Atlases
- •6.4.3 Statistical Atlases
- •6.4.3.1 Anatomical Variability Handling
- •6.4.3.2 Recent Works
- •References
- •7.1 Introduction
- •7.2 Linear Structure Detection Methods
- •7.3.1 CCM for Imaging Diabetic Peripheral Neuropathy
- •7.3.2 CCM Image Characteristics and Noise Artifacts
- •7.4.1 Foreground and Background Adaptive Models
- •7.4.2 Local Orientation and Parameter Estimation
- •7.4.3 Separation of Nerve Fiber and Background Responses
- •7.4.4 Postprocessing the Enhanced-Contrast Image
- •7.5 Quantitative Analysis and Evaluation of Linear Structure Detection Methods
- •7.5.1 Methodology of Evaluation
- •7.5.2 Database and Experiment Setup
- •7.5.3 Nerve Fiber Detection Comparison Results
- •7.5.4 Evaluation of Clinical Utility
- •7.6 Conclusion
- •References
- •8.1 Introduction
- •8.2 Methods
- •8.2.1 Linear Feature Detection by MDNMS
- •8.2.2 Check Intensities Within 1D Window
- •8.2.3 Finding Features Next to Each Other
- •8.2.4 Gap Linking for Linear Features
- •8.2.5 Quantifying Branching Structures
- •8.3 Linear Feature Detection on GPUs
- •8.3.1 Overview of GPUs and Execution Models
- •8.3.2 Linear Feature Detection Performance Analysis
- •8.3.3 Parallel MDNMS on GPUs
- •8.3.5 Results for GPU Linear Feature Detection
- •8.4.1 Architecture and Implementation
- •8.4.2 HCA-Vision Features
- •8.4.3 Linear Feature Detection and Analysis Results
- •8.5 Selected Applications
- •8.5.1 Neurite Tracing for Drug Discovery and Functional Genomics
- •8.5.2 Using Linear Features to Quantify Astrocyte Morphology
- •8.5.3 Separating Adjacent Bacteria Under Phase Contrast Microscopy
- •8.6 Perspectives and Conclusions
- •References
- •9.1 Introduction
- •9.2 Bone Imaging Modalities
- •9.2.1 X-Ray Projection Imaging
- •9.2.2 Computed Tomography
- •9.2.3 Magnetic Resonance Imaging
- •9.2.4 Ultrasound Imaging
- •9.3 Quantifying the Microarchitecture of Trabecular Bone
- •9.3.1 Bone Morphometric Quantities
- •9.3.2 Texture Analysis
- •9.3.3 Frequency-Domain Methods
- •9.3.4 Use of Fractal Dimension Estimators for Texture Analysis
- •9.3.4.1 Frequency-Domain Estimation of the Fractal Dimension
- •9.3.4.2 Lacunarity
- •9.3.4.3 Lacunarity Parameters
- •9.3.5 Computer Modeling of Biomechanical Properties
- •9.4 Trends in Imaging of Bone
- •References
- •10.1 Introduction
- •10.1.1 Adolescent Idiopathic Scoliosis
- •10.2 Imaging Modalities Used for Spinal Deformity Assessment
- •10.2.1 Current Clinical Practice: The Cobb Angle
- •10.2.2 An Alternative: The Ferguson Angle
- •10.3 Image Processing Methods
- •10.3.1 Previous Studies
- •10.3.2 Discrete and Continuum Functions for Spinal Curvature
- •10.3.3 Tortuosity
- •10.4 Assessment of Image Processing Methods
- •10.4.1 Patient Dataset and Image Processing
- •10.4.2 Results and Discussion
- •10.5 Summary
- •References
- •11.1 Introduction
- •11.2 Retinal Imaging
- •11.2.1 Features of a Retinal Image
- •11.2.2 The Reason for Automated Retinal Analysis
- •11.2.3 Acquisition of Retinal Images
- •11.3 Preprocessing of Retinal Images
- •11.4 Lesion Based Detection
- •11.4.1 Matched Filtering for Blood Vessel Segmentation
- •11.4.2 Morphological Operators in Retinal Imaging
- •11.5 Global Analysis of Retinal Vessel Patterns
- •11.6 Conclusion
- •References
- •12.1 Introduction
- •12.1.1 The Progression of Diabetic Retinopathy
- •12.2 Automated Detection of Diabetic Retinopathy
- •12.2.1 Automated Detection of Microaneurysms
- •12.3 Image Databases
- •12.4 Tortuosity
- •12.4.1 Tortuosity Metrics
- •12.5 Tracing Retinal Vessels
- •12.5.1 NeuronJ
- •12.5.2 Other Software Packages
- •12.6 Experimental Results and Discussion
- •12.7 Summary and Future Work
- •References
- •13.1 Introduction
- •13.2 Volumetric Image Visualization Methods
- •13.2.1 Multiplanar Reformation (2D slicing)
- •13.2.2 Surface-Based Rendering
- •13.2.3 Volumetric Rendering
- •13.3 Volume Rendering Principles
- •13.3.1 Optical Models
- •13.3.2 Color and Opacity Mapping
- •13.3.2.2 Transfer Function
- •13.3.3 Composition
- •13.3.4 Volume Illumination and Illustration
- •13.4 Software-Based Raycasting
- •13.4.1 Applications and Improvements
- •13.5 Splatting Algorithms
- •13.5.1 Performance Analysis
- •13.5.2 Applications and Improvements
- •13.6 Shell Rendering
- •13.6.1 Application and Improvements
- •13.7 Texture Mapping
- •13.7.1 Performance Analysis
- •13.7.2 Applications
- •13.7.3 Improvements
- •13.7.3.1 Shading Inclusion
- •13.7.3.2 Empty Space Skipping
- •13.8 Discussion and Outlook
- •References
- •14.1 Introduction
- •14.1.1 Magnetic Resonance Imaging
- •14.1.2 Compressed Sensing
- •14.1.3 The Role of Prior Knowledge
- •14.2 Sparsity in MRI Images
- •14.2.1 Characteristics of MR Images (Prior Knowledge)
- •14.2.2 Choice of Transform
- •14.2.3 Use of Data Ordering
- •14.3 Theory of Compressed Sensing
- •14.3.1 Data Acquisition
- •14.3.2 Signal Recovery
- •14.4 Progress in Sparse Sampling for MRI
- •14.4.1 Review of Results from the Literature
- •14.4.2 Results from Our Work
- •14.4.2.1 PECS
- •14.4.2.2 SENSECS
- •14.4.2.3 PECS Applied to CE-MRA
- •14.5 Prospects for Future Developments
- •References
- •15.1 Introduction
- •15.2 Acquisition of DT Images
- •15.2.1 Fundamentals of DTI
- •15.2.2 The Pulsed Field Gradient Spin Echo (PFGSE) Method
- •15.2.3 Diffusion Imaging Sequences
- •15.2.4 Example: Anisotropic Diffusion of Water in the Eye Lens
- •15.2.5 Data Acquisition
- •15.3 Digital Processing of DT Images
- •15.3.2 Diagonalization of the DT
- •15.3.3 Gradient Calibration Factors
- •15.3.4 Sorting Bias
- •15.3.5 Fractional Anisotropy
- •15.3.6 Other Anisotropy Metrics
- •15.4 Applications of DTI to Articular Cartilage
- •15.4.1 Bovine AC
- •15.4.2 Human AC
- •References
- •Index
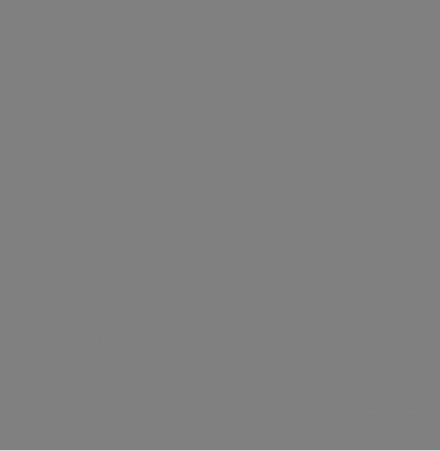
110 |
D.P. Costello and P.A. Kenny |
Fig. 5.13 (a) T1w GE image (b) global segmentation using the GMM and (c) classification of visceral fat
after global segmentation using the GMM. Region growing allows for classification based on spatial location. This is illustrated in Fig. 5.10 where both the liver and visceral fat are differentiated from fat in the image. Further work is required to fully automate classification.
5.6 Conclusions
This chapter reviewed the challenges associated with the quantification of fat in MR images. Accurate segmentation and analysis of fat using MRI is not a trivial matter. Ideally, MR images acquired for fat analysis should contain three distinct
5 Fat Segmentation in Magnetic Resonance Images |
111 |
tissue classes: fat, soft tissue and air. However, as a result of the many artifacts inherent to MRI this ideal image model is rarely attained. Therefore, consideration must be given to both the PVE and intensity inhomogeneities when segmenting fat in MR images. It is crucial that fat segmentation is approached with a thorough understanding of these artifacts and the limitations they present. A number of techniques used to segment fat in the presence of inhomogeneities were outlined in this chapter.
Image acquisition is the most important step in the quantification and analysis of fat using MRI. Before scanning patients, the imaging sequence should be optimized to achieve a balance between image contrast and acquisition time. Selection of an appropriate imaging sequence, such as the WS–bSSFP can significantly reduce the complexity of the segmentation algorithm required.
Fat classification currently requires manual intervention and will remain a significant challenge in the future. Future work in this field will investigate the prospect of fully automating the classification process and the use of soft segmentation algorithms involving fuzzy sets to overcome the PVE.
References
1.British Nutrition Foundation Obesity Task Force: Obesity: the report of the British Nutrition Foundation Task Force, John Wiley & Sons (1999)
2.Sarr´ıa, A., Moreno, L.A., et al.: Body mass index, triceps skinfold and waist circumference in screening for adiposity in male children and adolescents. Acta Pædiatrica 90(4), 387–392 (2001)
3.Peters, D., et al.: Estimation of body fat and body fat distribution in 11-year-old children using magnetic resonance imaging and hydrostatic weighing, skinfolds, and anthropometry. Am. J. Hum. Biol. 6(2), 237–243 (1994)
4.Rush, E.C., et al.: Prediction of percentage body fat from anthropometric measurements: comparison of New Zealand European and Polynesian young women. Am. J. Clin. Nutr. 66(1), 2–7 (1997)
5.(WHO), W.H.O. [cited; Available from:www.who.int/dietphysicalactivity/publication/facts/ obesity/en/. (2008)
6.Siegel, M.J., et al.: Total and intraabdominal fat distribution in preadolescents and adolescents: measurement with MR imaging. Radiology 242(3), 846–56 (2007)
7.Kullberg, J., et al.: Whole-body adipose tissue analysis: comparison of MRI, CT and dual energy X-ray absorptiometry. Br. J. Radiol. 82(974), 123–130 (2009)
8.Seidell, J.C., Bakker, C.J., van der Kooy, K.: Imaging techniques for measuring adipose-tissue distribution–a comparison between computed tomography and 1.5-T magnetic resonance. Am. J. Clin. Nutr. 51(6), 953–957 (1990)
9.Barnard, M.L., et al.: Development of a rapid and efficient magnetic resonance imaging technique for analysis of body fat distribution. NMR Biomed. 9(4), 156–64 (1996)
10.Thomas, E.L., et al.: Magnetic resonance imaging of total body fat. J. Appl. Physiol. 85(5), 1778–85 (1998)
11.Chan, Y.L., et al.: Body fat estimation in children by magnetic resonance imaging, bioelectrical impedance, skinfold and body mass index: a pilot study. J Paediatr. Child Health 34(1), 22–28 (1998)
12.Kamel, E.G., McNeill, G., Van Wijk, M.C.: Change in intra-abdominal adipose tissue volume during weight loss in obese men and women: correlation between magnetic resonance imaging and anthropometric measurements. Int. J. Obes. Relat. Metab. Disord. 24(5), 607–613 (2000)

112 |
D.P. Costello and P.A. Kenny |
13.Ross, R., et al.: Influence of diet and exercise on skeletal muscle and visceral adipose tissue in men. J. Appl. Physiol. 81(6), 2445–2455 (1996)
14.Terry, J.G., et al.: Evaluation of magnetic resonance imaging for quantification of intraabdominal fat in human beings by spin-echo and inversion-recovery protocols. Am. J. Clin. Nutr. 62(2), 297–301 (1995)
15.Brennan, D.D., et al.: Rapid automated measurement of body fat distribution from whole-body MRI. AJR Am. J. Roentgenol. 185(2), 418–23 (2005)
16.Peng, Q., et al.: Automated method for accurate abdominal fat quantification on water-saturated magnetic resonance images. J. Magn. Reson. Imaging. 26(3), 738–46 (2007)
17.Kovanlikaya, A., et al.: Fat quantification using three-point dixon technique: in vitro validation. Acad. Radiol. 12(5), 636–639 (2005)
18.Goyen, M.: In: Goyen, M. (ed.) Real Whole Body MRI Requirements, Indications, Perspectives, 1 edn., vol. 1, p. 184. Berlin, Mc Graw Hill (2007)
19.Peng, Q., et al.: Water-saturated three-dimensional balanced steady-state free precession for fast abdominal fat quantification. J. Magn. Reson. Imaging . 21(3), 263–271 (2005)
20.Warren, M., Schreiner, P.J., Terry, J.G.: The relation between visceral fat measurement and torso level–is one level better than another? The Atherosclerosis Risk in Communities Study, 1990–1992. Am. J. Epidemiol. 163(4), 352–358 (2006)
21.Dugas-Phocion, G., et al.: Improved EM-Based tissue segmentation and partial volume effect quantification in multi-sequence brain MRI. In: Lecture Notes in Computer Science, Medical
|
Image Computing and Computer-Assisted Intervention – MICCAI 2004, vol. 3216, p. 7. (2004) |
22. |
´ |
Gonz´alez Ballester, M.A., Zisserman, A.P. Brady, M.: Estimation of the partial volume effect |
|
|
in MRI. Med. Image Anal. 6(4), 389–405 (2002) |
23. |
Rajapakse, J.C., Kruggel, F.: Segmentation of MR images with intensity inhomogeneities. |
|
Image Vis. Comput. 16(3), 165–180 (1998) |
24. |
Li, X., et al.: Partial volume segmentation of brain magnetic resonance images based on |
|
maximum a posteriori probability. Med. Phys. 32(7), 2337–2345 (2005) |
25. |
Horsfield, M.A., et al.: Incorporating domain knowledge into the fuzzy connectedness frame- |
|
work: Application to brain lesion volume estimation in multiple sclerosis. Med. Imaging IEEE |
|
Trans. 26(12), 1670–1680 (2007) |
26. |
Siyal, M.Y., Yu, L.: An intelligent modified fuzzy c-means based algorithm for bias estimation |
|
and segmentation of brain MRI. Pattern Recognit. Lett. 26(13), 2052–2062 (2005) |
27. |
Yun, S., Kyriakos, W.E., et al.: Projection-based estimation and nonuniformity correction of |
|
sensitivity profiles in phased-array surface coils. J. Magn. Reson. Imaging. 25(3), 588–597 |
|
(2007) |
28. |
Murakami, J.W., Hayes, C.E. Weinberger, E. Intensity correction of phased-array surface coil |
|
images. Magn. Reson. Med. 35(4), 585–590 (1996) |
29. |
Pham, D.L., Prince, J.L.: An adaptive fuzzy C-means algorithm for image segmentation in the |
|
presence of intensity inhomogeneities. Pattern Recognit. Lett. 20(1), 57–68 (1999) |
30. |
Nie, S., Zhang, Y., Li, W., Chen, Z.: A novel segmentation method of MR brain images based |
|
on genetic algorithm. IEEE International Conference on Bioinformatics and Biomed. Eng. |
|
729–732 (2007) |
31. |
Wells, W.M., et al.: Adaptive segmentation of MRI data. Med. Imaging IEEE Trans. 15(4), |
|
429–442 (1996) |
32. |
Guillemaud, R.: Uniformity correction with homomorphic filtering on region of interest. |
|
In: Image Processing, 1998. ICIP 98. Proceedings. 1998 International Conference on (1998) |
33. |
Behrenbruch, C.P., et al.: Image filtering techniques for medical image post-processing: an |
|
overview. Br. J. Radiol. 77(suppl 2), S126–132 (2004) |
34.Guillemaud, R., Brady, M.: Estimating the bias field of MR images. Med. Imaging IEEE Trans. 16(3), 238–251 (1997)
35.Yang, G.Z., et al.: Automatic MRI adipose tissue mapping using overlapping mosaics. MAGMA 14(1), 39–44 (2002)
36.Leroy-Willig, A., et al.: Body composition determined with MR in patients with Duchenne muscular dystrophy, spinal muscular atrophy, and normal subjects. Magn. Reson. Imaging 15(7), 737–44 (1997)
5 Fat Segmentation in Magnetic Resonance Images |
113 |
37.Zhang, Y.J., Gerbrands, J.J.: Comparison of thresholding techniques using synthetic images and ultimate measurement accuracy. In: Pattern Recognition, 1992. Vol.III. Conference C: Image, Speech and Signal Analysis, Proceedings., 11th IAPR International Conference on (1992)
38.Mehmet, S., Bulent, S.: Survey over image thresholding techniques and quantitative performance evaluation. J. Electron. Imaging 13(1), 146–168 (2004)
39.Sonka, M., Hlavac, V., Boyle, R.: In: Hilda, G. (ed.) Image Processing, Analysis, and Machine Vision, International Student Edition, 3rd edn., vol. 1. Thomson, Toronto, p. 829. (2008)
40.Nualsawat, H., et al.: FASU: A full automatic segmenting system for ultrasound images. In: Proceedings of the Sixth IEEE Workshop on Applications of Computer Vision. 2002, IEEE Computer Society.
41.Otsu, N.: A threshold selection method from gray-level histograms. Syst. Man Cybern. IEEE Trans. 9(1), 62–66 (1979)
42.Sezgin, M., Sankur, B.: Survey over image thresholding techniques and quantitative performance evaluation. J. Electron. Imaging 13(1), 146–168 (2004)
43.Lee, H., Park, R.H.: Comments on ‘An optimal multiple threshold scheme for image segmentation’. Syst. Man Cybern. IEEE Trans. 20(3), 741–742 (1990)
44.Peng, Q., et al.: Automated method for accurate abdominal fat quantification on water-saturated magnetic resonance images. J. Magn. Reson. Imaging 26(3), 738–746 (2007)
45.Dempster, A.P., Laird, N.M., R.D. B.: Maximum likelihood from incomplete data via the EM algorithm. J. R. Stat. Soc. Ser. B (Methodological) 39(1), 1–38 (1977)
46.Lynch, M., et al.: Automatic seed initialization for the expectation-maximization algorithm and its application in 3D medical imaging. J. Med. Eng. Technol. 31(5), 332–340 (2007)
47.Liang, Z.: Tissue classification and segmentation of MR images. Eng. Med. Biol. Mag. IEEE. 12(1), 81–85 (1993)
48.Pham, D.L., Xu, C., Prince, J.L.: Current methods in medical image segmentation. Annu. Rev. Biomed. Eng. 2, 315–37 (2000)
49.Yan, K., Engelke, K., Kalender, W.A.: A new accurate and precise 3-D segmentation method for skeletal structures in volumetric CT data. Med. Imaging IEEE Trans. 22(5), 586–598 (2003)
50.Wee-Chung Liew, A., Yan, H., Yang, M.: Robust adaptive spot segmentation of DNA microarray images. Pattern Recogn. 36(5), 1251–1254 (2003)
51.Dougherty, G.: Digital Image Processing for Medical Applications, 1 edn., vol. 1, p. 447. Cambridge University Press, New York (2009)
52.Shen, W., et al.: Adipose tissue quantification by imaging methods: A proposed classification. Obes. Res. 11(1), 5–16 (2003)
53.Laharrague, P., Casteilla, L.: Bone Marow Adipose Tissue, 1 edn. Nutrition and health. Humana Press, New Jersey (2007)
54.Mantatzis, M., Prassopoulos P.: Total body fat, visceral fat, subcutaneous fat, bone marrow fat? What is important to measure? AJR Am. J. Roentgenol. 189(6), W386 (2007); author reply W385