
Emerging Tools for Single-Cell Analysis
.pdf
Acknowledgments |
47 |
We have identified factors that constrain the performance of cell sorters. The following conditions present a workable compromise. Using standard fluidic components, an operating pressure to about 690 N/m2 (100 psi, 7 atm) can be maintained. At that pressure, the jet velocity is 37 m/s. A simple lens system illuminates the jet with a 20-µm light spot. Cells suspended in the jet generate light pulses that are 1–2 µs wide. A 1-µs pulse separation sets the illumination cycle time at 2.5 µs. The number of events that can be handled with confidence (duty cycle of 0.1) is about 4 × 104 events/s. At 37 m/s, a 70-µm jet optimally forms drops at 118 kHz. The break-off time of the drops is approximately 30 drop periods. The break-off point can be kept stable to about one-eighth of a drop. Under these conditions, on average one in three drops contains a cell, with 24% of the drops containing a single event. If all drops with a wanted cell are sorted, accepting drops with multiple events, the instrument delivers samples of 85% purity. Enforcement of a coincidence exclusion zone of 1.5 drops yields better than 99% purity with a yield of 75%. A robust design limits downtime and allows for short start-up and shutdown protocols. Such instruments achieve effective throughput rates of 25,000 cells/s or a little under 108 cells/h.
A modest increase in speed of 1.5 times, at an increased risk of nozzle clogging, can be achieved by reducing the diameter of the jet. A jet with a diameter of 50 mm can be made to form drops at 165 kHz. The drop frequency may also be increased by increasing the pressure. However, the square root relationship between pressure and jet velocity limits the practical possibilities. In order to match the drop frequency with the current analysis rate, the pressure must be increased to ~104 N/m2. A pressure of 100 atm can be achieved only with technically sophisticated instrumentation and will negatively affect overall robustness. Furthermore, a higher jet velocity reduces the dwell time of the particle in the laser spot, requiring a higher illumination intensity and much faster electronics. Clearly, the current definition of high-speed sorting represents the practical limits of this technology.
Considering the technical challenges in further increasing sorter speed, parallel operation of several instruments is an attractive alternative. In the parallel approach, technical development concentrates on simplifying sorter design and the sharing of common resources by several instruments. Experimental prototypes of high-speed sorters that are much simpler and much more compact than the current commercial machines have been built in my laboratory. Two compact sorters need not occupy more than a bench that is 1.5 m wide. The instruments can be operated simultaneously by one technician. Two instruments double the effective yield to 2 × 108 cells/h. For critical samples, such as in the preparation of samples for clinical applications, a third sort head could be maintained in a stand-by mode to take over in case of catastrophic failure of one of the operating systems.
ACKNOWLEDGMENTS
This work has been supported by the Department of Energy, grant number DE-FG06- 93ER61662, and the Washington Technology Center. Research grants have been supplied by Becton Dickinson, Cytomation, Systemix, Pharmacopeia, and Sumitomo.

48 |
High-Speed Cell Sorting |
Assistance in kind has been provided by V-TEK and Applied Precision. Many students have helped me investigate aspects of flow cytometer design. Ellen Bosgoed, Hugo Gelevert, Maurie Brewer, and Jeannie Fehlauer deserve special mention. Chip Asbury is the senior junior scientist in my laboratory. All new ideas must pass his scrutiny. He has provided valuable help in the preparation of this chapter. The construction of experimental instruments is not possible without the efforts of a dedicated technical crew. Richard Esposito and Brian Wadey have been responsible for much of the design and construction of electronic and mechanical modules. Leah LaTray brought design errors to light, while skillfully sorting cells. I owe much to Barb Trask for a conscientious reading of the manuscript and numerous valuable suggestions.
REFERENCES
Baumgarth N, Parks DR, Bigos M, Stovel RT, Anderson MA, Gerstein RA, King UC, Jager GC, Herman OC, Herzenberg LA, Herzenberg LA (1998): Tracking green fluorescent protein transgene-expressing cells by nine-color FACS. Presentation at the Colorado Springs meeting of the International Society for Analytical Cytology. 28 February–5 March 1998.
Denny MW (1993): Air and Water. Princeton, NJ: Princeton University Press.
Gray JW, Dean PN, Fuscoe JC, Peters DC, Trask BJ, van den Engh GJ, Van Dilla MA (1987): High-speed chromosome sorting. Science 238, 323–329.
Kachel V, Menke E (1979): Hydrodynamic properties of flow cytometric instruments. In Melamed MR, Mullaney PF, Mendelsohn ML (eds). Flow Cytometry and Sorting. New York: Wiley.
Peters D, Branscomb E, Dean P, Merrill T, Pinkel D, Van Dilla M, Gray JW (1985): The LLNL high-speed sorter: Design features, operational characteristics, and biological utility. Cytometry 6:290–301.
Pinkel D, Stovel R (1985): Flow chambers and sample handling. In Dean PN (ed). Flow cytometry: Instrumentation and Data Analysis. New York: Academic Press.
Rayleigh JWS (1877): Theory of Sound. London: MacMillan. Reprinted 1945, New York: Dover.
Sasaki DT, Tichenore EH, Lopez F, Combs J, Uchida N, Smith C, Stokdijk W, Vardenega M, Buckle A-M, Chen B, Tushinski R, Tsukamoto AS, Hoffman R (1995): Development of a clinically applicable highspeed flow cytometer for the isolation of transplantable human hematopoietic stem cells. J Hematother 4:504–514.
Taylor JR (1997): An Introduction to Error Analysis. Sausolito, CA: University Science Books.
van den Engh GJ (1998): Particle separating apparatus and method, US Pat. 5,819,948.
van den Engh GJ (1999): Particle separating apparatus and method, US Pat. 6,003,678.
van den Engh GJ, Farmer C (1992): Photo-bleaching and photon saturation in flow cytometry. Cytometry 13:669–677.
van den Engh GJ, Stokdijk W (1989): Parallel processing data acquisition system for multi-laser flow cytometry and cell sorting. Cytometry 10, 282–293.
Van Dilla MA, Deaven LL, Albright KL, Allen NA, Bartholdi MF, Brown NC, Campbell EW, Carrano AV, Christensen M, Clark LM, Cram LS, Dean PN, de Jong P, Fawcett JJ, Fuscoe JC, Gray JW, Hildebrand CE, Jackson PJ, Jett JH, Killa S, Longmire JL, Lozes CR, Luedemann ML, McNinch JS, Mendelsohn ML, Meyne J, Meincke LJ, Moyzis RK, Mullikin J, Munk AC, Perlman J, Pederson L, Peters DC, Silva AJ, Trask BJ, van den Engh G (1990): The national laboratory gene library project. In Gray JW (ed). Flow Cytogenetics. London: Academic Press, pp 257–274.
Visser J, van den Engh G (1982): Immunofluorescence measurements by flow cytometry. In Wick G, Trail KN, Schauenstein K (eds). Immunofluorescence Technology. Amsterdam: Elsevier.
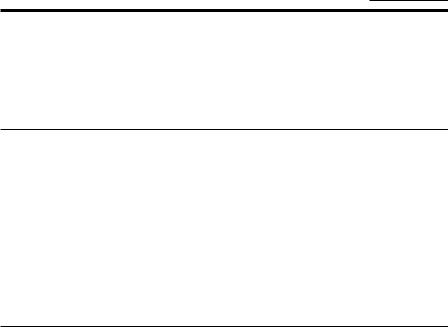
Emerging Tools for Single-Cell Analysis: Advances in Optical Measurement Technologies
Edited by Gary Durack, J. Paul Robinson Copyright © 2000 Wiley-Liss, Inc.
ISBNs: 0-471-31575-3 (Hardback); 0-471-22484-7 (Electronic)
3
Rare-Event Detection and Sorting
of Rare Cells
James F. Leary
University of Texas Medical Branch, Galveston, Texas
INTRODUCTION
Why Is the Analysis and Isolation of Rare Cells Important?
Just as in physics, where many of the simpler problems have been solved, biology is now entering a similar era. This is not to say that the major problems have been solved. Indeed, with the sequencing portion of the Human Genome Project about to be completed, a new era is about to begin. But many of the new biological problems of importance will involve looking beyond the simpler analyses of the past. For example, many of the new basic and clinical problems in biology will involve the detection, isolation, and molecular characterization of rare cells.
Rare cells can be defined as those of less than 0.1% frequency. Ultrarare cells can be defined as those of less than 0.001% frequency. These are terms for ease of discussion and are not universally agreed upon. In terms of flow cytometry and cell sorting, rare-cell applications not only push the limits of the technology but also require levels of staining specificity beyond those assumed by most biologists. It is important for engineers to understand that good technology can be made irrelevant by bad cell staining and preparation. The greatest difficulty with rare-cell applications is not the immediate technological constraints but the requirement that there be no weak links in the
49
50 |
Rare-Event Detection and Sorting of Rare Cells |
experimental methodology anywhere in the process from cell preparation, flow cytometry/cell sorting, and data analysis or subsequent analysis of isolated cells. Each step in the methodology must be excellent, and the entire process must be thought through to eliminate or deal with the weaker links of a given rare-cell application.
There are many rare-cell applications of importance to basic or clinical research. The ones discussed in this chapter help show the range not only of the applications but also of the technological challenges to engineers working in this field. Some basic research applications include (1) isolation of rare-cell clones with specific mutations or transfected genes, (2) isolation of clones with combinatorial libraries of inserted genes, and (3) studies of environmentally induced mutations in human cells. Some clinical applications include (1) isolation and sequence analysis of rare metastatic cells with mutated tumor suppressor genes for minimal residual disease monitoring and (2) isolation of rare human stem/progenitor cell subsets for autologous transplantation.
One of the major impediments of flow cytometry/cell-sorting technology is that biologists are unaware that many of the limitations of the currently available commercial instrumentation are due more to the lack of markets for manufacturers than to true technological limitations. Also, many biologists are unaware of what can be accomplished technologically with the instrumentation that they already have. Indeed, lack of a true understanding of the technology by many biology researchers has been one of the major reasons why many of the more challenging biological applications either are not being done or are being done poorly. Thus engineers may be puzzled or frustrated by the fact that even the present technology is not being fully utilized. While there are some fundamental technical limitations, as there are in all fields, few of these limits are as yet being reached by current commercial instrumentation. New technology must be “smart technology” that does not require considerable expertise of users who are frequently not well trained in the physical sciences. So the challenges to engineers are not only to push the technology but also to make something complex appear simple because it is implemented with smart technology that does not depend more than is absolutely necessary on the skill of the cell sorter operator. Unfortunately, due to the lack of smart technology in cell sorting, cell sorting remains an “art” that is not widespread. It has also led to the popularity of a number of alternative “simpler” cell separation technologies that appeal to users due to their simplicity, even though flow cytometry/cell sorting is considerably more powerful if it is properly used.
Why Are the Analysis and Isolation of Rare Cells Difficult?
There are a number of reasons why the analysis and isolation of rare cells are difficult. First, at the level of rare cells, few, if any, individual markers/probes for rare cells have adequate signal-to-noise (S/N) ratios. The main limitations for rare-cell analysis and sorting are not the S/N ratio of the instrument but rather the S/N ratio of the cell sample. Sometimes it is not possible to construct cell probes that have the specificity required by a given rare-cell application. But since flow cytometry and cell sorting approach the problem from the vantage point of individual cells, there are major pos-

Why Is It Important to Consider Theoretical Aspects of the Problem? |
51 |
sibilities for enhancement of S/N ratios by eliminating cells not-of-interest from the analysis. This can lead to overall S/N ratios that are many thousands of times better on single cells than they would be on populations of cells. For example, a probe may do a good job of labeling the rare cells of interest, but it may also stain another cell type not-of-interest. However, if the cells not-of-interest can be labeled with another probe that makes it possible to exclude them from the analysis, the first probe may be able to allow successful isolation of the rare cell when used in a Boolean logic of measured probe levels in cells, which eliminates the cells not-of-interest. Thus it is at least as important to have good negative markers/probes to eliminate as many as possible of the non–rare cells not-of-interest. As the cells become more rare, more markers/probes are frequently required, leading to the requirement for additional flow cytometric parameters. The true power of this technology is its ability to look at multiple probes on single cells. It is very unlikely for a single probe to be both sensitive and specific enough to detect rare cells, and almost never does a single probe work for ultrarare cells. Second, as cells become more rare, the sampling statistics problem can become more and more difficult. On the one hand, if total sample size is limited as in some applications, the researcher is forced to look for only a few cells in the entire sample. If, on the other hand, the total sample size is very large but the frequency of the rare cells is very low, a huge number of total cells must be examined. Unless the flow cytometer/cell sorter is very fast, the time required may be totally impractical or, in the case of the analysis of living cells, beyond the time limits imposed by either the stability of the parameter being measured or the viability of the cells. Third, due to the limitations of the preceding problems, there are necessarily trade-offs between yield and purity of the analyzed or sorted sample. This problem is starting to be addressed in commercial instrumentation, but only in some simple ways.
WHY IS IT IMPORTANT TO CONSIDER THEORETICAL ASPECTS OF THE PROBLEM?
It is important to consider as many of the theoretical aspects as possible to avoid getting into impractical or impossible situations. Unfortunately many engineers forget to take into account fundamental requirements of cells, particularly living cells, in their designs of flow cytometers/cell sorters. In this chapter many of these limitations, including those of the cells, will be taken into account to produce some technical specifications for the “ideal” instrument. Likewise, many biologists are so eager to perform a rare-cell analysis or sort that they fail to consider theoretical limitations imposed by instrument design and basic physics or by the rare-cell sampling statistics imposed by the given application. The problems of rare-cell analysis and cell sorting cover a large range of disciplines. Failure to address the full range of problems frequently results in failure of the particular application. The most difficult thing about rare-cell analysis and sorting is that everything has to work very well at the same time. If any aspect of the process is weak, the total effort frequently results in failure. Hence many of the partial or only average-quality solutions of non-rare-cell applications do not work for rare-cell applications. For example, small amounts of nonspe-

52 |
Rare-Event Detection and Sorting of Rare Cells |
cific staining are frequently not important for non-rare-cell applications. But a 1% or even a 0.1% nonspecific staining background (in terms of numbers of false-positive staining cells) in an application involving rareor ultra-rare-cell subpopulations can make the application impossible unless ways can be found to reduce this nonspecific staining level to some acceptable level lower than the specific staining frequency (the frequency of true-positive cells).
SOME FUNDAMENTAL PROBLEMS IN RARE-CELL DETECTION AND ISOLATION
Statistical Sampling Limits
In the case of limited total sample size, there must be a sufficient number of cells analyzable to obtain statistically or biologically meaningful results. For example, if total sample size is limited and frequencies of rare cells that are analyzable are low, it may not be possible to analyze enough cells to be meaningful at either the statistical or biological level. Since most rare-cell experiments are difficult and costly, this should be taken into account before plunging mindlessly into an attempt to analyze or sort rare cells. Mathematical and statistical modeling of the situation is wise to do beforehand, as it may change the fundamental approach to the problem. When the number of rare cells is very small, it is important to use combinatorial rather than Poisson statistics as Poisson statistics are only an approximation that is not particularly stable below about 50 rare cells (Rosenblatt et al., 1997). For example, to ensure with 95% confidence that the single rare (10–6 frequency ) cell has been sorted actually requires that a minimum of nearly three million cells must be sampled. Since the probability of successfully isolating the rare cell of interest above false-positive background events becomes more difficult for rare cells, it is important to sample an even higher number than this because it is highly likely that there will be false-positive cells sorted. The times needed to isolate a given number of desired rare cells as a function of sorting rate are illustrated in Table 3.1 (from Rosenblatt et al., 1997).
If rare single cells are to be isolated for either cloning or single-cell polymerase chain reaction (PCR), the proper approach is to take these rare-cell sampling statistics into account and perform single-cell, multitube sorting. An important principle of rare-cell sorting is that at very rare cell levels perhaps no cell markers, for example, monoclonal antibodies, will be good enough to pull out pure populations of rare cells from a noisy background. But if rare cells are isolated by single-cell sorting, 100% pure rare cells can always be isolated. If the S/N ratio is very poor, the number of tubes of single isolated cells becomes impractical. But with modern molecular biology methods such as PCR, even single-copy DNA or small numbers of messenger RNA (mRNA) sequences from a single cell can be quickly expanded into 106–108 copies (Zhang et al., 1992). So the principle is to recover a single copy of the DNA or mRNA template from a sorted single cell and then make many, many copies of that template much more rapidly than can be accomplished even by high-speed cell sorting. The basic idea of single-cell, multitube cell sorting is shown in Figure 3.1. Obvi-

Some Fundamental Problems in Rare-Cell Detection and Isolation |
53 |
T A B L E 3.1. Time Required to Collect Desired Cells as Function of |
|
||||||
Sorting Ratea |
|
|
|
|
|
|
|
|
|
|
|
|
|
|
|
Desired Number |
|
|
Time (s) Required to Collect Desired Cells by |
|
|||
of Cells with |
Total Number |
|
|
||||
|
|
Sorting Rateb |
|
|
|||
Selected |
of Sort |
|
|
|
|
||
|
|
|
|
|
|
||
Properties |
Decisions |
2,500 |
5,000 |
10,000 |
20,000 |
50,000 |
100,000 |
|
|
|
|
|
|
|
|
1 |
2,990,000 |
1,196 |
598 |
299 |
150 |
60 |
30 |
10 |
15,705,214 |
6,282 |
3,141 |
1,570 |
785 |
314 |
157 |
100 |
116,997,126 |
46,799 |
23,399 |
11,700 |
5,850 |
2,340 |
1,170 |
1000 |
1,052,577,091 |
421,031 |
210,515 |
105,258 |
52,629 |
21,052 |
10,526 |
aFrequency of cells with selected characteristics = 10–6 (0.95 level of assurance). bIn sort decisions per second.
ously cells can be sorted into tube arrays, or multiwell plates. The flow cytometric information associated with each sorted cell can remain associated with all the subsequent molecular measurements by a method known in the field as indexed cell sorting (Stovel and Sweet, 1979). We have combined flow cytometric indexed cellsorting data with molecular data in hybrid files that we have then used for subsequent analysis with multivariate statistical packages such as S-Plus for Windows.
Our practical experience agrees quite well with these theoretical predictions. When we have attempted to isolate rare (10–6 frequency) cells (labeled with very good probes!), we have found that we usually get 2 to 3 rare cells successfully isolated per 10 single-cell sorts, as depicted in Figure 3.1. Similarly, we have had applications where the probes are not very good and the combination of limited sampling statistics due to low numbers of cells available and poor probes have made impossible the successful isolation of less rare cells of a frequency of 10–5. So one must pay careful attention to whether enough cells are available to reach proper sampling statistics and whether the probes are good enough to practically isolate the rare cells of interest.
Rare-Event Detection Is Usually Only Possible with Multiple Probes
While many markers/probes look very good in non-rare-cell applications, few markers/probes look good at the rare-cell level and very few at the level of ultrarare cells. It is important to have some idea of the S/N ratio of the marker/probe system being used before wasting considerable energies trying to do an impossible application. Simple mathematical modeling of data using each probe can be used to predict the S/N ratio of that probe. In most cases one should expect to use multiple probes to detect rare cells (Ryan et al., 1984). Ultra-rare-cell applications usually require a minimum of three to four parameters and frequently cocktails of probes designed to minimize the number of required acquisition parameters and to reduce data complexity. The receiver operating characteristic (ROC; Swets and Pickett, 1982; Beck and Shultz, 1986; Hanley, 1989; Choi, 1998 ) long familiar to many engineers but only beginning to be used in flow cytometry and cell sorting (Leary et al., 1997,

54 |
Rare-Event Detection and Sorting of Rare Cells |
F i g . 3.1. General principle of single-cell, multitube PCR analysis of sorted single cells is to obtain one or more copies of a DNA or mRNA template from a single sorted cell. Some of the singly sorted cells will be false positives due to the inability of specific marker probes to have a high enough S/N ratio above false-positive staining background.
1998a, b, 1999; Hokanson et al., 1999) can be used to predict the power of each probe, singly and in combination. A discussion of ROC analysis and an example appear later in this chapter.
Analysis Speeds Must Be Fast Enough to Be Practical
When the total sample size is large and the rare-cell frequency small, there may be some fundamental limits imposed by instrument stability/operation, marker stability, and cell viability. Thus there is usually a time window imposed by these variables that should be carefully considered when either designing an instrument for an application or attempting an application on an existing instrument. In most cases this time window will require an analysis speed in excess of 10,000 cells/s for rare-cell applications and an analysis speed in excess of 40,000 cells/s for ultra-rare-cell applications. In terms of fundamental limitations it is possible to build instruments capable of analysis speeds in excess of 100,000 cells/s, if properly designed. But to do efficient high-speed sorting of rare cells, it is wise to do the fundamental cell classification/sort decision in two stages. The first stage should classify most of the cells with easy decisions so that the second stage can spend more time making the tough decisions. Most of the time we know that the cells are not of interest; the decision is straightforward and can usually be accomplished by some simple Boolean combination of simple gates of the original parameters. But there will always be some poten-
Some Fundamental Problems in Rare-Cell Detection and Isolation |
55 |
tial false-positive cells that must be distinguished from the true-positive rare cells of interest. Making these tougher choices may require more elaborate gating and perhaps mathematical combinations of parameters (e.g., discriminant functions) that are more complicated and require more time to process the signals. One should also remember that mindless high-speed digitization of signals merely leads to a river of digital data that must be processed at least partially in real time. Otherwise, one would have to deal with the problem of storing and processing many gigabytes of data per sample.
Limits in Sorting Speeds and Purities
Sorting is fundamentally more difficult and slower than analysis for a number of reasons. First, sorting requires that the rare cells be properly classified, which itself is difficult. Cell sorting should really be considered as real-time data analysis. The calculations and classifications must be done fast enough to reach a sort decision, typically less than a millisecond after excitation of the cell. Fortunately there are new methods available to accomplish this feat (Corio and Leary, 1993, 1996; Leary et al., 1997, 1998, 1999). By using one or more linked, high-speed lookup tables, one can perform complex linear and nonlinear “calculations” at memory speeds, enabling their use for real-time statistical classifications suitable for cell-sorting decisions. The lookup tables are precalculated for a range of useful values based on data obtained from an aliquot of the cell sample, as is typically done for any cell-sorting decision. The incoming data are then compared to lookup table values that then can be used to issue a sort/no-sort decision.
Second, as any flow cytometrist knows, the Achilles heel of a flow cytometer is the flow; that is, the stability of the fluidics is fragile and difficult to maintain. Since high-speed fluidic switching is still under development and has not yet been applied to cell sorting, droplet sorting is presently the method of choice, although this may change in the not-too-distant future. While fluidic switching has problems of its own, it has many potential advantages over droplet sorting, not the least of which is the safety issue of sorting biohazardous materials in a closed system rather than in an open system with aerosol generation. Advances in microfluidics may well change this situation.
Not only is the break-off position of a cell sorter difficult to maintain, but as the number of cells per second is increased for sorting of rare cells, the stability becomes less reliable. The stickiness of the cells makes clumping a problem at high cell concentrations (e.g., greater than 5 × 107 cells/ml). In terms of the fluidics, the cells themselves start to contribute to the viscosity of the sample stream, making transport difficult and less predictable. As discussed later in more detail, a high-speed cell sorter for rare cells should be able to measure the arrival statistics of the cells to determine if the cell preparation is adequate to permit isolation of these rare cells. As discussed later in this chapter, cell arrival statistics is a critically important measure of cell sorter performance during actual cell sorting of rare cells. If the fluidics are working properly, cell arrival statistics should be random. Cell arrival statistics can be described by simple queuing theory (Gross and Harris, 1985) familiar to many engineers, and
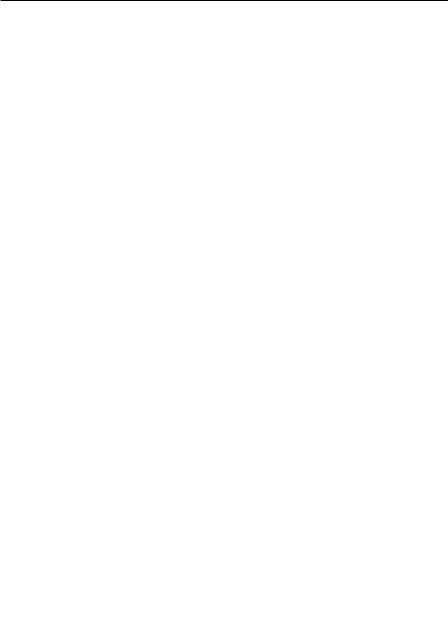
56 |
Rare-Event Detection and Sorting of Rare Cells |
discussed in the flow cytometry/cell-sorting field in a number of papers by different groups (Lindmo et al., 1981; van Rotterdam et al., 1992; Leary, 1994).
IMPORTANCE OF MODELING IN RARE-CELL ANALYSIS
Simple One-Parameter Modeling
The simplest modeling involves either constructing actual data mixtures of negative, non–rare cells and positive, rare cells or constructing mathematical models of these subpopulations with mathematical curves with known properties (Cupp et al., 1984). If pure populations of each cell type are available, it is possible to “tag” the data points so that when a data mixture is made, the yield and purity of rare cells in a sort gate can be modeled. If such data are not available, the cell subpopulations can be parametrically modeled, most easily but not always correctly, with Gaussians. This mathematical approach leads to easy statistical analysis of yields and purities, including probabilities of misclassification, a subject to be explored in greater depth later in this chapter.
Multiparameter Modeling and the Use of “Tagged” Data Mixtures
As the data become more complex, methods have been developed that permit easy modeling of multiparameter flow cytometric data (Redelman and Coder, 1994; Leary et al., 1996). Basically, each cell in a listmode flow cytometry data file is tagged with an additional classification parameter that is not used in the subsequent analysis process other than testing the validity of the classification process at the very end. An example of this cell-tagging process in a datafile is shown in Figure 3.2 (Leary et al., 1997).
F i g . 3.2. Tagged parameter is an additional correlated listmode parameter that is not used directly in the data analysis routine being used but instead serves as a truth table for the correct identity of each cell.