
- •Preface
- •Contents
- •Chapter 1
- •1.1 International Financial Markets
- •Foreign Exchange
- •Covered Interest Parity
- •Uncovered Interest Parity
- •Futures Contracts
- •1.2 National Accounting Relations
- •National Income Accounting
- •The Balance of Payments
- •1.3 The Central Bank’s Balance Sheet
- •Chapter 2
- •2.1 Unrestricted Vector Autoregressions
- •Lag-Length Determination
- •Granger Causality, Econometric Exogeniety and Causal
- •Priority
- •The Vector Moving-Average Representation
- •Impulse Response Analysis
- •Forecast-Error Variance Decomposition
- •Potential Pitfalls of Unrestricted VARs
- •2.2 Generalized Method of Moments
- •2.3 Simulated Method of Moments
- •2.4 Unit Roots
- •The Levin—Lin Test
- •The Im, Pesaran and Shin Test
- •The Maddala and Wu Test
- •Potential Pitfalls of Panel Unit-Root Tests
- •2.6 Cointegration
- •The Vector Error-Correction Representation
- •2.7 Filtering
- •The Spectral Representation of a Time Series
- •Linear Filters
- •The Hodrick—Prescott Filter
- •Chapter 3
- •The Monetary Model
- •Cassel’s Approach
- •The Commodity-Arbitrage Approach
- •3.5 Testing Monetary Model Predictions
- •MacDonald and Taylor’s Test
- •Problems
- •Chapter 4
- •The Lucas Model
- •4.1 The Barter Economy
- •4.2 The One-Money Monetary Economy
- •4.4 Introduction to the Calibration Method
- •4.5 Calibrating the Lucas Model
- •Appendix—Markov Chains
- •Problems
- •Chapter 5
- •Measurement
- •5.2 Calibrating a Two-Country Model
- •Measurement
- •The Two-Country Model
- •Simulating the Two-Country Model
- •Chapter 6
- •6.1 Deviations From UIP
- •Hansen and Hodrick’s Tests of UIP
- •Fama Decomposition Regressions
- •Estimating pt
- •6.2 Rational Risk Premia
- •6.3 Testing Euler Equations
- •Volatility Bounds
- •6.4 Apparent Violations of Rationality
- •6.5 The ‘Peso Problem’
- •Lewis’s ‘Peso-Problem’ with Bayesian Learning
- •6.6 Noise-Traders
- •Problems
- •Chapter 7
- •The Real Exchange Rate
- •7.1 Some Preliminary Issues
- •7.2 Deviations from the Law-Of-One Price
- •The Balassa—Samuelson Model
- •Size Distortion in Unit-Root Tests
- •Problems
- •Chapter 8
- •The Mundell-Fleming Model
- •Steady-State Equilibrium
- •Exchange rate dynamics
- •8.3 A Stochastic Mundell—Fleming Model
- •8.4 VAR analysis of Mundell—Fleming
- •The Eichenbaum and Evans VAR
- •Clarida-Gali Structural VAR
- •Appendix: Solving the Dornbusch Model
- •Problems
- •Chapter 9
- •9.1 The Redux Model
- •9.2 Pricing to Market
- •Full Pricing-To-Market
- •Problems
- •Chapter 10
- •Target-Zone Models
- •10.1 Fundamentals of Stochastic Calculus
- •Ito’s Lemma
- •10.3 InÞnitesimal Marginal Intervention
- •Estimating and Testing the Krugman Model
- •10.4 Discrete Intervention
- •10.5 Eventual Collapse
- •Chapter 11
- •Balance of Payments Crises
- •Flood—Garber Deterministic Crises
- •11.2 A Second Generation Model
- •Obstfeld’s Multiple Devaluation Threshold Model
- •Bibliography
- •Author Index
- •Subject Index

2.5. PANEL UNIT-ROOT TESTS |
59 |
This is called a parametric bootstrap because the error terms are drawn from the parametric normal distribution. An alternative is to do a nonparametric bootstrap. Here, you resample the estimated residuals, which are in a sense, the data. To do a nonparametric bootstrap, do the following. Estimate (2.83) using the data. Denote the OLS residuals by
(ˆ²11, ²ˆ21, . . . , ²ˆN1) |
← obs. 1 |
(ˆ²12, ²ˆ22, . . . , ²ˆN2) |
← obs. 2 |
. |
. |
. |
. |
. |
. |
(ˆ²1T , ²ˆ2T , . . . , ²ˆNT ) |
← obs. T |
Now resample the residual vectors with replacement. For each observation t = 1, . . . , T, draw one of the T possible residual vectors with probability T1 . Because the entire vector is being resampled, the crosssectional correlation observed in the data is preserved. Let the resampled vectors be
(² |
, ² |
, . . . , ² |
) |
← |
obs. 1 |
||
11 |
21 |
|
N1 |
|
|
|
|
(² |
, ² |
, . . . , ² |
) |
|
obs. 2 |
||
12 |
22 |
. |
N2 |
|
|
← . |
|
|
|
. |
|
|
|
|
. |
|
|
. |
|
|
|
|
. |
(² |
, ² |
, . . . , ² |
|
) |
← |
obs. T |
|
1T |
2T |
|
NT |
|
|
and use these resampled residuals to build up values of ∆qit recursively
ˆ
using (2.83) with µˆi and φij, and run the Levin-Lin test on these observations but do not subtract o the cross-sectional mean, and do not make the τ adjustments. This gives a realization of τ. Now repeat a large number of times to get the nonparametric bootstrap distribution of τ.
The Im, Pesaran and Shin Test
Im, Pesaran and Shin suggest a very simple panel unit root test. They begin with the ADF representation (2.72) for individual i (reproduced
here for convenience) |
(eq. 2.84)(35) |
ki |
|
jX |
|
∆q˜it = αi + δit + βiq˜it−1 + φij∆q˜it−j + ²it, |
(2.84) |
=1 |
|
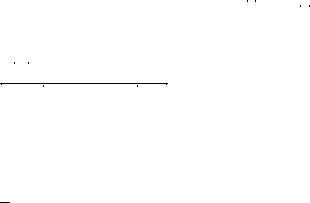
|
60 |
CHAPTER 2. SOME USEFUL TIME-SERIES METHODS |
||||||||||||
|
where E(² |
|
common time e ect may be |
|||||||||||
(36) |
|
it²js) = 0, i 6= j for all t, s. A |
|
N |
|
|
||||||||
removed in which case q˜it = qit − (1/N) |
|
j=1 qjt is the deviation from |
||||||||||||
|
the cross-sectional average as the basic |
unit of analysis. |
|
|
||||||||||
|
P |
|
|
|
|
|
|
|||||||
|
|
Let τi be the studentized coe cient from the ith ADF regression. |
||||||||||||
|
Since the ²it are assumed to be independent across individuals, the τi are |
|||||||||||||
|
|
|
|
|
|
|
|
|
|
|
1 |
|
N |
|
|
|
|
|
|
|
|
|
|
|
|
||||
|
also independent, and by the central limit theorem, τNT = |
|
|
i=1 τi |
||||||||||
(37) |
N |
|
||||||||||||
|
|
|
|
|
|
|
|
|
|
|
|
then as |
||
|
converges to the standard normal distribution Þrst as T → ∞ P |
|
||||||||||||
|
N → ∞. That is |
|
|
|
|
|
|
|
|
|||||
|
|
√ |
|
|
|
|
|
|
|
|
|
|
|
|
|
|
|
N[¯τNT − E(τit|βi = 0)] |
|
D N(0, 1), |
(2.85) |
||||||||
|
|
|
|
|
|
|
|
|
||||||
|
|
|
|
qVar(τit|β = 0) |
|
|
→ |
|
|
as T → ∞, N → ∞. IPS report selected critical values for τ¯NT with the conditional mean and variance adjustments of the distribution. A selected set of these critical values are reproduced in Table 2.5. An alternative to relying on the asymptotic distribution is to do a residual bootstrap of the τNT statistic. As before, when doing the bootstrap, do not subtract o the cross-sectional mean.
The Im, Pesaran and Shin test as well as the Maddala—Wu test (discussed below) relax the homogeneity restrictions under the alternative hypothesis. Here, the null hypothesis
H0 : β1 = · · · = βN = β = 0,
is tested against the alternative
HA : β1 < 0 β2 < 0 · · · ββN < 0.
The alternative hypothesis is not H0, which is less restrictive than the Levin—Lin alternative hypothesis.
The Maddala and Wu Test
Maddala and Wu [99] point out that the IPS strategy of combining independent tests to construct a joint test is an idea suggested by R.A. Fisher [53]. Maddala and Wu follow Fisher’s suggestion and propose following test. Let the p-value of τi from the augmented Dickey—Fuller
test for a unit root be pi = Prob(τ < τi) = R τi f(x)dx be the p-
−∞
value of τi from the ADF test on (2.72), where f(τ) is the probability

2.5. PANEL UNIT-ROOT TESTS |
|
|
61 |
||||||||
Table 2.5: Selected Exact Critical Values for the IPS τ¯NT Statistic |
|||||||||||
|
|
|
|
|
|
|
|
|
|
|
|
|
|
|
|
|
|
|
|
|
|
|
|
|
|
|
|
|
Constant |
|
|
Trend |
|
|
|
|
|
|
|
|
|
|
|
|
|
|
|
|
|
|
T |
20 |
40 |
100 |
20 |
40 |
100 |
|
|
|
|
|
|
|
|
|
|
|
|
|
|
|
|
|
|
|
|
|
|
|
|
||
|
|
|
|
|
A. 5 percent |
|
|
|
|
||
|
|
|
5 |
-2.19 |
-2.16 |
-2.15 |
-2.82 |
-2.77 |
-2.75 |
|
|
|
|
|
10 |
-1.99 |
-1.98 |
-1.97 |
-2.63 |
-2.60 |
-2.58 |
|
|
|
|
N |
15 |
-1.91 |
-1.90 |
-1.89 |
-2.55 |
-2.52 |
-2.51 |
|
|
|
|
|
20 |
-1.86 |
-1.85 |
-1.84 |
-2.49 |
-2.48 |
-2.46 |
|
|
|
|
|
25 |
-1.82 |
-1.81 |
-1.81 |
-2.46 |
-2.44 |
-2.43 |
|
|
|
|
|
|
|
|
|
|
|
|
|
|
|
|
|
|
|
B. 10 percent |
|
|
|
|
||
|
|
|
|
|
|
|
|
|
|
|
|
|
|
|
5 |
-2.04 |
-2.02 |
-2.01 |
-2.67 |
-2.63 |
-2.62 |
|
|
|
|
|
10 |
-1.89 |
-1.88 |
-1.88 |
-2.52 |
-2.50 |
-2.49 |
|
|
|
|
N |
15 |
-1.82 |
-1.81 |
-1.81 |
-2.46 |
-2.44 |
-2.44 |
|
|
|
|
|
20 |
-1.78 |
-1.78 |
-1.77 |
-2.42 |
-2.41 |
-2.40 |
|
|
|
|
|
25 |
-1.75 |
-1.75 |
-1.75 |
-2.39 |
-2.38 |
-2.38 |
|
|
|
|
|
|
|
|
|
|
|
|
|
|
|
|
|
|
|
|
|
|
|
|
|
|
Source: Im, Pesaran and Shin [78].
density function of τ. Solve for g(p), the density of pi by the method of transformations, g(pi) = f(τi)|J| where J = dτi/dpi is the Jacobian of the transformation, and |J| is its absolute value. Since dpi/dτi = f(τi), the Jacobian is 1/f(τi) and g(pi) = 1 for 0 ≤ pi ≤ 1. That is, pi is uniformly distributed on the interval [0, 1] (pi U[0, 1]).
Next, let yi = −2 ln(pi). Again, using the method of transformations, the probability density function of yi is h(yi) = g(pi)|dpi/dyi|. But g(pi) = 1 and |dpi/dyi| = pi/2 = (1/2)e−yi/2, so it follows that h(yi) = (1/2)e−yi/2 which is the chi-square distribution with 2 degrees of freedom. Under cross-sectional independence of the error terms ²it, the joint test statistic also has a chi-square distribution
N |
|
Xi |
|
λ = −2 ln(pi) χ22N . |
(2.86) |
=1 |
|
The asymptotic distribution of the IPS test statistic was established by sequential T → ∞, N → ∞ asymptotics, which some econometri-

62 CHAPTER 2. SOME USEFUL TIME-SERIES METHODS
cians view as being too restrictive.26 Levin and Lin derive the asymptotic distribution of their test statistic by allowing both N and T simultaneously to go to inÞnity. A remarkable feature of the Maddala—Wu— Fisher test is that it avoids issues of sequential or joint N, T asymptotics. (2.86) gives the exact distribution of the test statistic.
The IPS test is based on the sum of τi, whereas the Maddala—Wu test is based on the sum of the log p-values of τi. Asymptotically, the two tests should be equivalent, but can di er in Þnite samples. Another advantage of Maddala—Wu is that the test statistic distribution does not depend on nuisance parameters, as does IPS and LL. The disadvantage is that p-values need to be calculated numerically.
Potential Pitfalls of Panel Unit-Root Tests
Panel unit-root tests need to be applied with care. One potential pitfall with panel tests is that the rejection of the null hypothesis does not mean that all series are stationary. It is possible that out of N timeseries, only 1 is stationary and (N-1) are unit root processes. This is an example of a mixed panel. Whether we want the rejection of the unit root process to be driven by a single outlier or not depends on the purpose the researcher uses the test.27
A second potential pitfall is that cross-sectional independence is a regularity condition for these tests. Transforming the observations by subtracting o the cross-sectional means will leave some residual dependence across individuals if common time e ects are generated by a multi-factor process. This residual cross-sectional dependence can potentially generate errors in inference.
A third potential pitfall concerns potential small sample size distortion of the tests. While most of the attention has been aimed at
26That is, they deduce the limiting behavior of the test statistic Þrst by letting T → ∞ holding N Þxed, then letting N → ∞ and invoking the central limit theorem.
27Bowman [17] shows that both the LL and IPS tests have low power against outlier driven alternatives. He proposes a test that has maximal power. Taylor and Sarno [131] propose a test based on Johansen’s [80] maximum likelihood approach that can test for the number of unit-root series in the panel. Computational considerations, however, generally limit the number of time-series that can be analyzed to 5 or less.