
- •Preface
- •Contents
- •Chapter 1
- •1.1 International Financial Markets
- •Foreign Exchange
- •Covered Interest Parity
- •Uncovered Interest Parity
- •Futures Contracts
- •1.2 National Accounting Relations
- •National Income Accounting
- •The Balance of Payments
- •1.3 The Central Bank’s Balance Sheet
- •Chapter 2
- •2.1 Unrestricted Vector Autoregressions
- •Lag-Length Determination
- •Granger Causality, Econometric Exogeniety and Causal
- •Priority
- •The Vector Moving-Average Representation
- •Impulse Response Analysis
- •Forecast-Error Variance Decomposition
- •Potential Pitfalls of Unrestricted VARs
- •2.2 Generalized Method of Moments
- •2.3 Simulated Method of Moments
- •2.4 Unit Roots
- •The Levin—Lin Test
- •The Im, Pesaran and Shin Test
- •The Maddala and Wu Test
- •Potential Pitfalls of Panel Unit-Root Tests
- •2.6 Cointegration
- •The Vector Error-Correction Representation
- •2.7 Filtering
- •The Spectral Representation of a Time Series
- •Linear Filters
- •The Hodrick—Prescott Filter
- •Chapter 3
- •The Monetary Model
- •Cassel’s Approach
- •The Commodity-Arbitrage Approach
- •3.5 Testing Monetary Model Predictions
- •MacDonald and Taylor’s Test
- •Problems
- •Chapter 4
- •The Lucas Model
- •4.1 The Barter Economy
- •4.2 The One-Money Monetary Economy
- •4.4 Introduction to the Calibration Method
- •4.5 Calibrating the Lucas Model
- •Appendix—Markov Chains
- •Problems
- •Chapter 5
- •Measurement
- •5.2 Calibrating a Two-Country Model
- •Measurement
- •The Two-Country Model
- •Simulating the Two-Country Model
- •Chapter 6
- •6.1 Deviations From UIP
- •Hansen and Hodrick’s Tests of UIP
- •Fama Decomposition Regressions
- •Estimating pt
- •6.2 Rational Risk Premia
- •6.3 Testing Euler Equations
- •Volatility Bounds
- •6.4 Apparent Violations of Rationality
- •6.5 The ‘Peso Problem’
- •Lewis’s ‘Peso-Problem’ with Bayesian Learning
- •6.6 Noise-Traders
- •Problems
- •Chapter 7
- •The Real Exchange Rate
- •7.1 Some Preliminary Issues
- •7.2 Deviations from the Law-Of-One Price
- •The Balassa—Samuelson Model
- •Size Distortion in Unit-Root Tests
- •Problems
- •Chapter 8
- •The Mundell-Fleming Model
- •Steady-State Equilibrium
- •Exchange rate dynamics
- •8.3 A Stochastic Mundell—Fleming Model
- •8.4 VAR analysis of Mundell—Fleming
- •The Eichenbaum and Evans VAR
- •Clarida-Gali Structural VAR
- •Appendix: Solving the Dornbusch Model
- •Problems
- •Chapter 9
- •9.1 The Redux Model
- •9.2 Pricing to Market
- •Full Pricing-To-Market
- •Problems
- •Chapter 10
- •Target-Zone Models
- •10.1 Fundamentals of Stochastic Calculus
- •Ito’s Lemma
- •10.3 InÞnitesimal Marginal Intervention
- •Estimating and Testing the Krugman Model
- •10.4 Discrete Intervention
- •10.5 Eventual Collapse
- •Chapter 11
- •Balance of Payments Crises
- •Flood—Garber Deterministic Crises
- •11.2 A Second Generation Model
- •Obstfeld’s Multiple Devaluation Threshold Model
- •Bibliography
- •Author Index
- •Subject Index
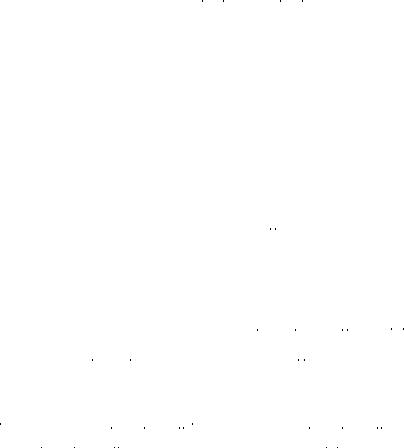
308 |
CHAPTER 10. TARGET-ZONE MODELS |
all variables except interest rates are in logarithms. The time derivative of a function x(t) is denoted with the ‘dot’ notation, xú (t) = dx(t)/dt. In order to work with these models, you need some background in stochastic calculus.
10.1Fundamentals of Stochastic Calculus
Let x(t) be a continuous-time deterministic process that grows at the constant rate, η such that, dx(t) = ηdt. Let G(x(t), t) be some possibly time-dependent continuous and di erentiable function of x(t). From calculus, you know that the total di erential of G is
dG = |
∂G |
dx(t) + |
∂G |
dt. |
(10.1) |
|
|
||||
|
∂x |
∂t |
|
If x(t) is a continuous-time stochastic process, however, the formula for the total di erential (10.1) doesn’t work and needs to be modiÞed. In particular, we will be working with a continuous-time stochastic process x(t) called a di usion process where the growth rate of x(t) randomly deviates from η,
dx(t) = ηdt + σdz(t). |
(10.2) |
ηdt is the expected change in x conditional on information available at t, σdz(t) is an error term and σ is a scale factor. z(t) is called a Wiener
process or Brownian motion and it evolves according to, |
|
||
√ |
|
|
|
z(t) = u t, |
(10.3) |
iid
where u N(0, 1). At each instant, z(t) is hit by an independent draw u from the standard normal distribution. InÞnitesimal changes in z(t)
can be thought of as |
|
√ |
|
|
|
|
|
|
√ |
|
|
√ |
|
|
||
|
|
|
|
|
|
|
|
|
|
|
|
|
|
|||
dz(t) = z(t + dt) − z(t) = ut+dt t + dt − ut |
|
t = u˜ |
|
dt, (10.4) |
||||||||||||
√ |
|
|
|
|
|
|
√ |
|
|
|
|
|
|
|
|
|
where ut+dt t + dt N(0, t |
+ dt) and u |
t |
|
|
t |
|
N(0, t) deÞne the new |
|||||||||
1 |
|
|
|
|
|
|
|
|
|
|
|
|||||
random variable u˜ N(0, 1). |
|
The di usion process is the continuous- |
time analog of the random walk with drift η. Sampling the di usion
|
|
|
|
|
|
|
|
|
|
|
|
√ |
|
|
|
√ |
|
|
1 |
|
|
|
√ |
|
|
|
√ |
|
|
|
|||||||
Since E[ut+dt |
|
|
|
t] = 0, and Var[ut+dt |
|
|
|
|
||||||||||
|
|
t + dt−ut |
|
t + dt−ut t] = t+dt−t = dt, |
||||||||||||||
|
√ |
|
|
√ |
|
|
|
|
|
|
√ |
|
|
|
|
iid |
||
|
|
|
|
|
|
|
|
|
|
|
|
|
||||||
ut+dt |
t + dt − ut |
|
t deÞnes a new random variable, u˜ |
dt, where u˜ N(0, 1). |

10.1. FUNDAMENTALS OF STOCHASTIC CALCULUS |
309 |
|||
x(t) at discrete points in time yields |
|
|
|
|
x(t + 1) − x(t) = |
Zt t+1 dx(s) |
|
|
|
= η Zt t+1 ds + σ Zt t+1 dz(s) |
(10.5) |
|||
= |
η + σu˜. |
|z(t {z− |
} |
|
|
|
+1) |
z(t) |
|
If x(t) follows the di usion process (10.2), it turns out that the total di erential of G(x(t), t) is
|
∂G |
|
∂G |
σ2 ∂2G |
|
||||
dG = |
|
dx(t) + |
|
dt + |
|
|
|
dt. |
(10.6) |
∂x |
|
|
2 |
||||||
|
|
∂t |
2 ∂x |
|
This result is known as Ito’s lemma. The next section gives a nonrigorous derivation of Ito’s lemma and can be skipped by uninterested readers.
Ito’s Lemma
Consider a random variable X with Þnite mean and variance, and a positive number θ > 0. Chebyshev’s inequality says that the probability that X deviates from its mean by more than θ is bounded by its variance divided by θ2
P{|X − E(X)| ≥ θ} ≤ |
Var(X) |
|
|
|
. |
(10.7) |
|
θ2 |
If z(t) follows the Wiener process (10.3), then E[dz(t)] = 0 and Var[dz(t)2] = E[dz(t)2] −[Edz(t)]2 = dt. Apply Chebyshev’s inequality to dz(t)2, to get
P{|[dz(t)]2 − E[dz(t)]2| > θ} ≤ (dtθ2)2 .
Since dt is a fraction, as dt → 0, (dt)2 goes to zero even faster than dt does. Thus the probability that dz(t)2 deviates from its mean dt becomes negligible over inÞnitesimal increments of time. This suggests
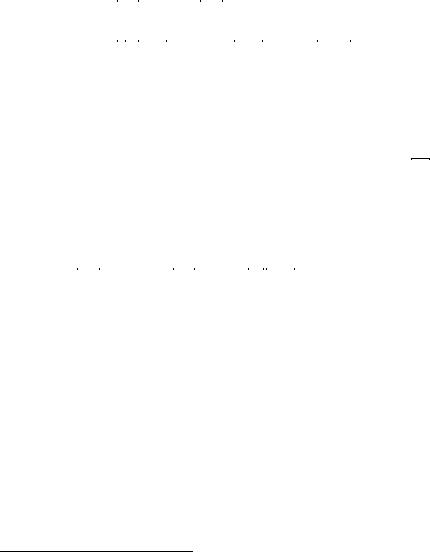
310 |
CHAPTER 10. TARGET-ZONE MODELS |
that you can treat the deviation of dz(t)2 from its mean dt as an error term of order O(dt2).2 Write it as
dz(t)2 = dt + O(dt2).
Taking a second-order Taylor expansion of G(x(t), t) gives
∆G = |
|
∂G |
∆x(t) + |
∂G |
∆t |
|
|
|
||
|
∂x |
∂t |
∆t2 |
+ 2∂x∂t[∆x(t)∆t]# |
||||||
|
|
|
|
|
||||||
+ 2 " |
∂x2 ∆x(t)2 + ∂t2 |
|||||||||
|
1 |
|
∂2G |
|
|
∂2G |
|
∂2G |
||
+ |
O(∆t2), |
|
|
|
|
(10.8) |
where O(∆t2) are the ‘higher-ordered’ terms involving (∆t)k with k > 2. You can ignore those terms when you send ∆t → 0.
If x(t) evolves according to the di usion process, you√know that ∆x(t) = η∆t + σ∆z(t), with ∆z(t) = u ∆t, and (∆x)2 = η2(∆t)2 + σ2(∆z)2 + 2ησ(∆t)(∆z) = σ2∆t + O(∆t3/2). Substitute these expressions into the square-bracketed term in (10.8) to get,
|
∂G |
|
∂G |
σ2 ∂2G |
(∆t) + O(∆t3/2). (10.9) |
||||
∆G = |
|
|
(∆x(t)) + |
|
(∆t) + |
|
|
|
|
∂x |
|
2 |
|||||||
|
|
∂t |
2 ∂x |
|
As ∆t → 0, (10.9) goes to (10.6), because the O(∆t3/2) terms can be ignored. The result is Ito’s lemma.
10.2The Continuous—Time Monetary Model
A deterministic setting. To see how the monetary model works in continuous time, we will start in a deterministic setting. As in chapter 3, all variables except interest rates are in logarithms. The money market equilibrium conditions at home and abroad are
m(t) − p(t) |
= |
φy(t) − αi(t), |
(10.10) |
m (t) − p (t) |
= |
φy (t) − αi (t). |
(10.11) |
2An O(dt2) term divided by dt2 is constant.
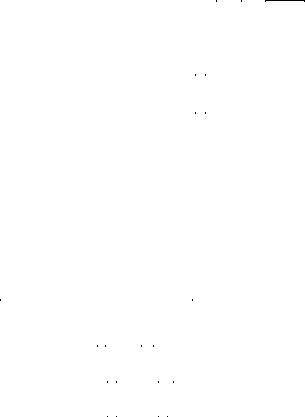
10.2. THE CONTINUOUS—TIME MONETARY MODEL |
311 |
International asset-market equilibrium is given by uncovered interest parity
i(t) − i (t) = sú(t). |
(10.12) |
The model is completed by invoking PPP |
|
s(t) + p (t) = p(t). |
(10.13) |
Combining (10.10)-(10.13) you get |
|
s(t) = f(t) + αsú(t), |
(10.14) |
where f(t) ≡ m(t) − m (t) − φ[y(t) − y (t)] are the monetary-model ‘fundamentals.’ Rewrite (10.14) as the Þrst-order di erential equation
sú(t) |
− |
s(t) |
|
= |
−f(t) |
. |
(10.15) |
|
α |
|
|||||||
|
|
α |
|
|||||
The solution to (10.15) is3 |
|
|
|
|
|
|
|
|
s(t) = α Zt ∞ e(t−x)/αf(x)dx |
|
|||||||
|
1 |
|
|
|
|
|
|
|
= |
1 |
et/α Zt |
∞ e−x/αf(x)dx. |
(10.16) |
||||
|
||||||||
α |
A stochastic setting. The stochastic continuous-time monetary model is
|
|
|
|
m(t) − p(t) |
= |
φy(t) − αi(t), |
(10.17) |
|||||||||
|
|
|
|
m (t) − p (t) |
= |
φy (t) − αi (t), |
(10.18) |
|||||||||
|
|
|
|
i(t) − i (t) |
= Et[sú(t)], |
|
(10.19) |
|||||||||
|
|
|
|
s(t) + p (t) |
= |
p(t). |
|
(10.20) |
||||||||
|
|
|
|
|
|
|
|
|
|
|
||||||
3To verify that (10.16) is a solution, take its time derivative |
α−2et/α |
|||||||||||||||
sú(t) = α et/α ·dt |
Zt |
|
e−x/αf(x)dx¸ + ·Zt |
e−x/αf(x)dx¸ |
||||||||||||
1 |
|
|
|
d |
|
|
|
∞ |
|
∞ |
|
|
||||
|
|
|
1 |
|
|
|
1 |
|
et/α Zt |
∞ |
|
|
|
|||
= − |
|
f(t) + |
|
|
|
e−x/αf(x)dx |
|
|
||||||||
α |
α2 |
|
|
|||||||||||||
= − |
1 |
f(t) + |
1 |
s(t) |
|
|
|
|
||||||||
|
|
|
|
|
|
|
||||||||||
α |
|
α |
|
|
|
|
Therefore, (10.16) solves (10.15).
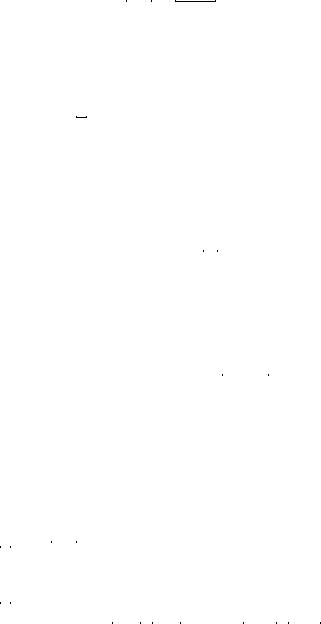
312 |
CHAPTER 10. TARGET-ZONE MODELS |
||||||||
Combine (10.17)-(10.20) to get |
|
|
|
|
|
|
|
||
E |
[sú(t)] |
− |
s(t) |
= |
−f(t) |
, |
(10.21) |
||
α |
|
α |
|||||||
t |
|
|
|
|
which is a Þrst-order stochastic di erential equation. To solve (10.21), mimic the steps used to solve the deterministic model to get the continuoustime version of the present-value formula
|
1 |
∞ e(t−x)/αEt[f(x)]dx. |
|
s(t) = |
α Zt |
(10.22) |
To evaluate the expectations in (10.22) you must specify the stochastic process governing the fundamentals. For this purpose, we assume that the fundamentals process follow the di usion process
df(t) = ηdt + σdz(t), |
(10.23) |
|
√ |
|
|
where η and σ are constants, and dz(t) = u dt is the standard Wiener process. It follows that
f(x) − f(t) = |
Zt x df(r)dr |
|
|
|
|
|
= |
Z x ηdr + Z x σdz(r) |
|
||||
|
t |
t |
q |
|
|
|
= |
η(x − t) + σu |
|
(x − t). |
(10.24) |
Take expectations of (10.24) conditional on time t information to get the prediction rule
Et[f(x)] = f(t) + η(x − t), |
(10.25) |
and substitute (10.25) into (10.22) to obtain
s(t) = α Zt ∞ e |
α |
[f(t) + η(x − t)]dx |
|
|
|
|
|
|
|
|
|||||||||
|
1 |
|
|
(t−x) |
|
|
|
|
|
|
|
|
|
|
|
|
|
|
|
= α |
|
e (f − ηt) Zt |
e− |
dx +ηe |
Zt |
xe− |
|
dx |
|||||||||||
1 |
|
t/α |
|
|
|
∞ x/α |
|
|
t/α |
∞ |
x/α |
|
|
||||||
|
|
|
|
|
|
|
|
|
|
|
|
|
|
|
|
|
|
|
|
|
|
|
|
|
|
|
|
a |
|
|
|
|
|
b |
|
|
|
||
|
|
|
|
|
| |
|
|
{z |
|
|
} |
| |
|
|
{z |
|
|
|
} |
= αη + f(t), |
(10.26) |