
- •Preface
- •Contents
- •Chapter 1
- •1.1 International Financial Markets
- •Foreign Exchange
- •Covered Interest Parity
- •Uncovered Interest Parity
- •Futures Contracts
- •1.2 National Accounting Relations
- •National Income Accounting
- •The Balance of Payments
- •1.3 The Central Bank’s Balance Sheet
- •Chapter 2
- •2.1 Unrestricted Vector Autoregressions
- •Lag-Length Determination
- •Granger Causality, Econometric Exogeniety and Causal
- •Priority
- •The Vector Moving-Average Representation
- •Impulse Response Analysis
- •Forecast-Error Variance Decomposition
- •Potential Pitfalls of Unrestricted VARs
- •2.2 Generalized Method of Moments
- •2.3 Simulated Method of Moments
- •2.4 Unit Roots
- •The Levin—Lin Test
- •The Im, Pesaran and Shin Test
- •The Maddala and Wu Test
- •Potential Pitfalls of Panel Unit-Root Tests
- •2.6 Cointegration
- •The Vector Error-Correction Representation
- •2.7 Filtering
- •The Spectral Representation of a Time Series
- •Linear Filters
- •The Hodrick—Prescott Filter
- •Chapter 3
- •The Monetary Model
- •Cassel’s Approach
- •The Commodity-Arbitrage Approach
- •3.5 Testing Monetary Model Predictions
- •MacDonald and Taylor’s Test
- •Problems
- •Chapter 4
- •The Lucas Model
- •4.1 The Barter Economy
- •4.2 The One-Money Monetary Economy
- •4.4 Introduction to the Calibration Method
- •4.5 Calibrating the Lucas Model
- •Appendix—Markov Chains
- •Problems
- •Chapter 5
- •Measurement
- •5.2 Calibrating a Two-Country Model
- •Measurement
- •The Two-Country Model
- •Simulating the Two-Country Model
- •Chapter 6
- •6.1 Deviations From UIP
- •Hansen and Hodrick’s Tests of UIP
- •Fama Decomposition Regressions
- •Estimating pt
- •6.2 Rational Risk Premia
- •6.3 Testing Euler Equations
- •Volatility Bounds
- •6.4 Apparent Violations of Rationality
- •6.5 The ‘Peso Problem’
- •Lewis’s ‘Peso-Problem’ with Bayesian Learning
- •6.6 Noise-Traders
- •Problems
- •Chapter 7
- •The Real Exchange Rate
- •7.1 Some Preliminary Issues
- •7.2 Deviations from the Law-Of-One Price
- •The Balassa—Samuelson Model
- •Size Distortion in Unit-Root Tests
- •Problems
- •Chapter 8
- •The Mundell-Fleming Model
- •Steady-State Equilibrium
- •Exchange rate dynamics
- •8.3 A Stochastic Mundell—Fleming Model
- •8.4 VAR analysis of Mundell—Fleming
- •The Eichenbaum and Evans VAR
- •Clarida-Gali Structural VAR
- •Appendix: Solving the Dornbusch Model
- •Problems
- •Chapter 9
- •9.1 The Redux Model
- •9.2 Pricing to Market
- •Full Pricing-To-Market
- •Problems
- •Chapter 10
- •Target-Zone Models
- •10.1 Fundamentals of Stochastic Calculus
- •Ito’s Lemma
- •10.3 InÞnitesimal Marginal Intervention
- •Estimating and Testing the Krugman Model
- •10.4 Discrete Intervention
- •10.5 Eventual Collapse
- •Chapter 11
- •Balance of Payments Crises
- •Flood—Garber Deterministic Crises
- •11.2 A Second Generation Model
- •Obstfeld’s Multiple Devaluation Threshold Model
- •Bibliography
- •Author Index
- •Subject Index

2.6. COINTEGRATION |
63 |
improving the power of unit root tests, Schwert [126] shows that there are regions of the parameter space under which the size of the augmented Dickey—Fuller test is wrong in small samples. Since the panel tests are based on the augmented Dickey—Fuller test in some way or another, it is probably the case that this size distortion will get impounded into the panel test. To the extent that size distortion is an issue, however, it is not a problem that is speciÞc to the panel tests.
2.6Cointegration
The unit root processes {qt} and {ft} will be cointegrated if there exists a linear combination of the two time-series that is stationary. To understand the implications of cointegration, let’s Þrst look at what happens when the observations are not cointegrated.
No cointegration. Let ξqt = ξqt−1 + uqt and ξft |
= ξft−1 + uft be |
(38) |
||
|
|
iid |
|
(39) |
two independent random walk processes where uqt N(0, σq2) and |
|
|||
iid |
|
|
|
|
uft N(0, σf2). Let zt = (zqt, zft)0 follow a stationary bivariate pro- |
(40) |
|||
cess such as a VAR. The exact process for zt doesn’t need to explicitly |
|
|
||
modeled at this point. Now consider the two unit root series built up |
|
|
||
from these components |
|
|
|
|
qt |
= ξqt + zqt, |
|
|
|
ft |
= ξft + zft. |
(2.87) |
|
|
Since qt and ft are driven by independent random walks, they will drift arbitrarily far apart from each other over time. If you try to Þnd a value of β to form a stationary linear combination of qt and ft, you will fail because
qt − βft = (ξqt − βξft) + (zqt − βzft). |
(2.88) |
For any value of β, ξqt −βξft = (˜u1 + u˜2 + · · · u˜t) where u˜t ≡ uqt −βuft so the linear combination is itself a random walk. qt and ft clearly do not share a long run relationship. There may, however, be short-run interactions between their Þrst di erences
à ∆ft |
! = |
à ∆zft |
! + |
à ²ft |
! . |
(2.89) |
∆qt |
|
∆zqt |
|
²qt |
|
|
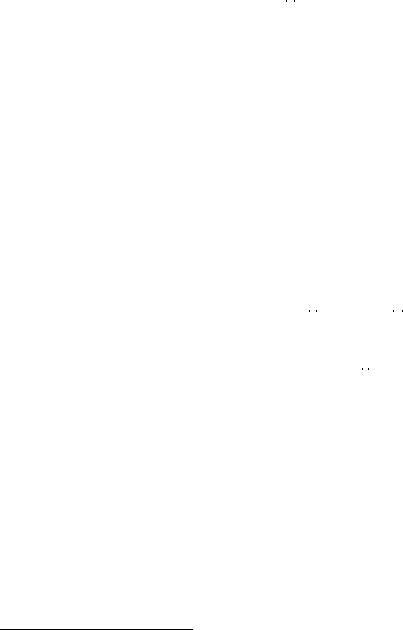
64 CHAPTER 2. SOME USEFUL TIME-SERIES METHODS
By analogy to the derivation of (2.58), if zt follows a Þrst-order VAR, you can show that (2.89) follows a vector ARMA process. Thus, when both {qt} and {ft} are unit root processes but are driven by independent random walks, they can be Þrst di erenced to induce stationarity and their Þrst di erences modeled as a stationary vector process.
Cointegration. {qt} and {ft} will be cointegrated if they are driven by
|
iid |
|
the same random walk, ξt = ξt−1 +²t, where ²t N(0, σ2). For example |
||
if |
|
|
qt |
= ξt + zqt, |
|
ft |
= φ(ξt + zft), |
(2.90) |
and you look for a value of β that renders |
|
|
qt − βft = (1 − βφ)ξt + zqt − βφzft, |
(2.91) |
stationary, you will succeed by choosing β = φ1 since qt − fφt = zqt − zft is the di erence between two stationary processes so it will itself be stationary. {qt} and {ft} share a long-run relationship. We say that they are cointegrated with cointegrating vector (1, −φ1 ). Since random walks are sometimes referred to as stochastic trend processes, when two series are cointegrated we sometimes say that they share a common trend.28
The Vector Error-Correction Representation
Recall that for the univariate AR(2) process, you can rewrite qt = ρ1qt−1 + ρ2qt−2 + ut in augmented Dickey—Fuller test equation form as
∆qt = (ρ1 + ρ2 − 1)qt−1 − ρ2∆qt−1 + ut, |
(2.92) |
iid |
+ ρ2 −1) = 0, |
where ut N(0, σu2). If qt is a unit root process, then (ρ1 |
and (ρ1 +ρ2 −1)−1 clearly doesn’t exist. There is in a sense a singularity
28Suppose you are analyzing three variables (q1t, q2t, q3t). If they are cointegrated, there can be at most 2 independent random walks driving the series. If there are 2 random walks, there can be only 1 cointegrating vector. If there is only 1 random walk, there can be as many as 2 cointegrating vectors.
2.6. COINTEGRATION |
65 |
in qt−1 because ∆qt is stationary and this can be true only if qt−1 drops out from the right side of (2.92).
By analogy, suppose that in the bivariate case the vector (qt, ft) is generated according to
" ft |
# |
= " a21 |
a22 |
# " |
ft−1 |
#+" b21 |
b22 |
# " |
ft−2 |
#+" |
uft |
# , (2.93) |
|||||||
qt |
|
a11 |
a12 |
|
|
qt 1 |
|
b11 |
b12 |
|
qt 2 |
|
uqt |
|
|
||||
|
|
|
|
|
|
|
|
− |
|
|
|
|
− |
|
|
|
|
|
|
where (uqt, uft)0 |
iid |
|
|
|
|
|
|
|
|
|
|
|
|
|
|
|
|
||
N(0, Σu). Rewrite (2.93) as the vector analog of the |
|||||||||||||||||||
augmented Dickey—Fuller test equation |
|
# " ∆ft−1 |
# + |
" uft |
# , |
||||||||||||||
" ∆ft |
# = " r21 |
r22 |
# " ft−1 |
# − " |
b21 |
b22 |
|||||||||||||
∆qt |
r11 |
r12 |
|
|
qt 1 |
|
b11 |
b12 |
|
∆qt 1 |
|
|
uqt |
|
|||||
|
|
|
|
|
|
|
|
− |
|
|
|
|
|
− |
|
|
(2.94) |
||
|
|
|
|
|
|
|
|
|
|
|
|
|
|
|
|
|
|
||
where |
|
" r21 |
|
# " |
|
|
|
|
|
|
|
1 # ≡ |
|
|
|
||||
|
|
r22 |
a21 |
+ b21 |
a22 + b22 |
|
|
|
|
||||||||||
|
|
r11 |
r12 |
|
= |
|
a11 |
+ b11 − 1 a12 |
+ b12 |
|
|
R. |
|
||||||
|
|
|
|
|
|
|
|
|
|
|
|
|
|
− |
|
|
|
|
|
If {qt} and {ft} are unit root processes, their Þrst di erences are stationary. This means the terms on the right hand side of (2.94) are stationary. Linear combinations of levels of the variables appear in the system. r11qt−1 + r12ft−1 appears in the equation for ∆qt and r21qt−1 + r22ft−1 appears in the equation for ∆ft.
If {qt} and {ft} do not cointegrate, there are no values of the rij coe cients that can be found to form stationary linear combinations of qt and ft. The level terms must drop out. R is the null matrix, and (∆qt, ∆ft) follows a vector autoregression.
If {qt} and {ft} do cointegrate, then there is a unique combination of the two variables that is stationary. The levels enter on the right side but do so in the same combination in both equations. This means that the columns of R are linearly dependent and the R, which is singular,
can be written as |
R = |
" r21 |
−βr21 |
# . |
|
|
|
|
||
|
|
|
|
r11 |
βr11 |
|
|
|
|
|
(2.94) can now be written as |
|
− |
|
|
|
|
|
|||
|
|
|
# " ∆ft−1 |
# + |
" uft |
# |
||||
" ∆ft |
# = |
" r21 |
# (qt−1 − βft−1) − " b21 |
b22 |
||||||
∆qt |
|
r11 |
|
|
b11 |
b12 |
∆qt 1 |
|
uqt |
|
−

66 CHAPTER 2. SOME USEFUL TIME-SERIES METHODS
= |
" r21 |
# zt−1 |
− |
" b21 |
b22 |
# " ∆ft−1 |
# + |
" uft |
# , (2.95) |
|
r11 |
|
|
b11 |
b12 |
∆qt 1 |
|
uqt |
|
−
(41)(eq.2.95)
(42)
(43)(eq.2.96)
where zt−1 ≡ qt−1 −βft−1 is called the error-correction term, and (2.95) is the vector error correction representation (VECM). A VAR in Þrst di erences would be misspeciÞed because it omits the error correction term.
To express the dynamics governing zt, multiply the equation for ∆ft by β and subtract the result from the equation for ∆qt to get
zt = (1 + r11 − βr21)zt−1 − (b11 + βb21)∆qt−1
−(b12 + βb22)∆ft−1 + uqt − βuft.
(44)(eq.2.97) The entire system is then given by
∆ft |
|
= |
b21 |
|
b22 |
r12 |
|
||
|
∆qt |
|
|
b11 |
|
b12 |
r11 |
βr21 |
|
zt |
(b11 + βb21) |
|
(b12 + βb22) 1 + r11 |
|
|||||
|
|
|
− |
|
− |
|
|
− |
|
+ |
|
uft |
. |
|
|
uqt |
uqt |
|
|
|
βuft |
|||
|
|
− |
|
|
(2.96)
|
|
∆qt−1∆ft−1
zt−1
(2.97)
|
(∆qt, ∆ft, zt)0 is a stationary vector, and (2.97) looks like a VAR(1) in |
||||||
|
these three variables, except that the columns of the coe cient matrix |
||||||
|
are linearly dependent. In many applications, the cointegration vector |
||||||
|
(1, −β) is given a priori by economic theory and does not need to be |
||||||
|
estimated. In these situations, the linear dependence of the VAR (2.97) |
||||||
|
tells you that all of the information contained in the VECM is preserved |
||||||
|
in a bivariate VAR formed with zt and either ∆qt or ∆ft. |
||||||
(45) |
Suppose you follow this strategy. To get the VAR for (∆qt, zt), |
||||||
substitute ft−1 = (qt−1 − zt−1)/β into the equation for ∆qt to get |
|||||||
|
∆qt = b11∆qt−1 + b12∆ft−1 + r11zt−1 + uqt |
||||||
|
|
= a11∆qt−1 + a12zt−1 + a13zt−2 + uqt, |
|||||
(46) |
where a11 = b11 + |
b12 |
, a12 = r11 − |
b12 |
, and a13 = |
b12 |
. Similarly, substitute |
β |
β |
β |
|||||
|
ft−1 out of the equation for zt to get |
zt = a21∆qt−1 + a22zt−1 + a23zt−2 + (uqt − βuft),