
- •Preface
- •Contents
- •Chapter 1
- •1.1 International Financial Markets
- •Foreign Exchange
- •Covered Interest Parity
- •Uncovered Interest Parity
- •Futures Contracts
- •1.2 National Accounting Relations
- •National Income Accounting
- •The Balance of Payments
- •1.3 The Central Bank’s Balance Sheet
- •Chapter 2
- •2.1 Unrestricted Vector Autoregressions
- •Lag-Length Determination
- •Granger Causality, Econometric Exogeniety and Causal
- •Priority
- •The Vector Moving-Average Representation
- •Impulse Response Analysis
- •Forecast-Error Variance Decomposition
- •Potential Pitfalls of Unrestricted VARs
- •2.2 Generalized Method of Moments
- •2.3 Simulated Method of Moments
- •2.4 Unit Roots
- •The Levin—Lin Test
- •The Im, Pesaran and Shin Test
- •The Maddala and Wu Test
- •Potential Pitfalls of Panel Unit-Root Tests
- •2.6 Cointegration
- •The Vector Error-Correction Representation
- •2.7 Filtering
- •The Spectral Representation of a Time Series
- •Linear Filters
- •The Hodrick—Prescott Filter
- •Chapter 3
- •The Monetary Model
- •Cassel’s Approach
- •The Commodity-Arbitrage Approach
- •3.5 Testing Monetary Model Predictions
- •MacDonald and Taylor’s Test
- •Problems
- •Chapter 4
- •The Lucas Model
- •4.1 The Barter Economy
- •4.2 The One-Money Monetary Economy
- •4.4 Introduction to the Calibration Method
- •4.5 Calibrating the Lucas Model
- •Appendix—Markov Chains
- •Problems
- •Chapter 5
- •Measurement
- •5.2 Calibrating a Two-Country Model
- •Measurement
- •The Two-Country Model
- •Simulating the Two-Country Model
- •Chapter 6
- •6.1 Deviations From UIP
- •Hansen and Hodrick’s Tests of UIP
- •Fama Decomposition Regressions
- •Estimating pt
- •6.2 Rational Risk Premia
- •6.3 Testing Euler Equations
- •Volatility Bounds
- •6.4 Apparent Violations of Rationality
- •6.5 The ‘Peso Problem’
- •Lewis’s ‘Peso-Problem’ with Bayesian Learning
- •6.6 Noise-Traders
- •Problems
- •Chapter 7
- •The Real Exchange Rate
- •7.1 Some Preliminary Issues
- •7.2 Deviations from the Law-Of-One Price
- •The Balassa—Samuelson Model
- •Size Distortion in Unit-Root Tests
- •Problems
- •Chapter 8
- •The Mundell-Fleming Model
- •Steady-State Equilibrium
- •Exchange rate dynamics
- •8.3 A Stochastic Mundell—Fleming Model
- •8.4 VAR analysis of Mundell—Fleming
- •The Eichenbaum and Evans VAR
- •Clarida-Gali Structural VAR
- •Appendix: Solving the Dornbusch Model
- •Problems
- •Chapter 9
- •9.1 The Redux Model
- •9.2 Pricing to Market
- •Full Pricing-To-Market
- •Problems
- •Chapter 10
- •Target-Zone Models
- •10.1 Fundamentals of Stochastic Calculus
- •Ito’s Lemma
- •10.3 InÞnitesimal Marginal Intervention
- •Estimating and Testing the Krugman Model
- •10.4 Discrete Intervention
- •10.5 Eventual Collapse
- •Chapter 11
- •Balance of Payments Crises
- •Flood—Garber Deterministic Crises
- •11.2 A Second Generation Model
- •Obstfeld’s Multiple Devaluation Threshold Model
- •Bibliography
- •Author Index
- •Subject Index
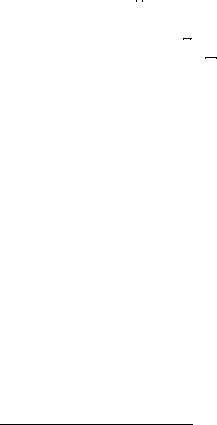
2.1. UNRESTRICTED VECTOR AUTOREGRESSIONS |
29 |
The e ect on q1t at time k of a one standard deviation orthogonalized innovation in η1 at time 0, is b11,k. Similarly, the e ect on q2k is b21,k. Graphing the transformed moving-average coe cients is an e cient method to examine the impulse responses.
You may also want to calculate standard error bands for the impulse responses. You can do this using the following parametric bootstrap procedure.5 Let T be the number of time-series observations you have
and let a ‘tilde’ denote pseudo values generated by the computer, then (12) ‘tilde’
ˆ
1. Take T + M independent draws from the N(0, Σ) to form the vector series {²˜t}.
2. Set startup values of qt at their mean values of 0 then recursively generate the sequence {q˜t} of length T + M according to (2.1)
using the estimated Aj matrices.
3. Drop the Þrst M observations to eliminate dependence on starting values. Estimate the simulated VAR. Call the estimated coe -
˜
cients Aj.
˜˜ ˜−1˜
4.Form the matrices Bj = CjΛ S. You now have one realization (13) of the parametric bootstrap distribution of the impulse response function.
5.Repeat the process say 5000 times. The collection of observations
˜
on the Bj forms the bootstrap distribution. Take the standard deviation of the bootstrap distribution as an estimate of the standard error.
Forecast-Error Variance Decomposition
In (2.7), you have decomposed q1t into orthogonal components. The innovation η1t is attributed to q1t and the innovation η2t is attributed
5The bootstrap is a resampling scheme done by computer to estimate the underlying probability distribution of a random variable. In a parametric bootstrap the observations are drawn from a particular probability distribution such as the normal. In the nonparametric bootstrap, the observations are resampled from the data.
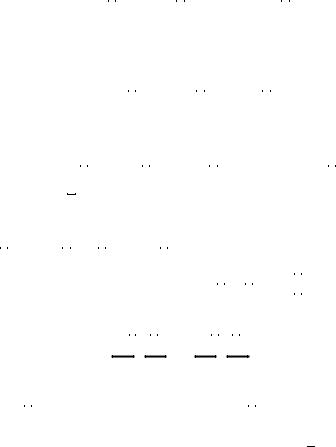
30 CHAPTER 2. SOME USEFUL TIME-SERIES METHODS
to q2t. You may be interested in estimating how much of the underlying variability in q1t is due to q1t innovations and how much is due to q2t innovations. For example, if q1t is a real variable like the log real exchange rate and q2t is a nominal quantity such as money and you might want to know what fraction of log real exchange rate variability is attributable to innovations in money. In the VAR framework, you can ask this question by decomposing the variance of the k-step ahead forecast error into contributions from the separate orthogonal components. At t + k, the orthogonalized and standardized moving-average representation is
q |
t+k = B0 |
η |
t+k + · · · + Bk |
η |
t + · · · |
(2.9) |
|
|
|
|
Take expectations of both sides of (2.9) conditional on information available at time t to get
Etqt+k = Bkηt + Bk+1ηt−1 + · · · |
(2.10) |
Now subtract (2.10) from (2.9) to get the k-period ahead forecast error vector
q |
t+k − Et |
q |
t+k = B0 |
η |
t+k + · · · + Bk−1 |
η |
t+1. |
(2.11) |
|
|
|
|
|
Because the ηt are serially uncorrelated and have covariance matrix I, the covariance matrix of these forecast errors is
E[qt+k − Etqt+k][qt+k − Etqt+k]0 |
= B0B00 + B1B10 + · · · + Bk−1Bk0 −1 |
||||||
= BjBj0 = b1,j, b2,j |
|
à b01,j |
! |
||||
k |
|
k |
³ |
|
´ |
b0 |
|
X |
|
X |
|
2,j |
|
||
j=0 |
|
j=0 |
|
|
|||
k |
|
k |
|
|
|
|
|
X |
b1,jb10 |
jX |
b2,jb20 |
|
|
|
|
= |
,j + |
,j, |
|
|
(2.12) |
||
j=0 |
({z |
=0 |
({zb) |
} |
|
|
|
| |
} | |
|
|
|
|||
|
a) |
|
|
|
|
|
|
where b1,j is the Þrst column of Bj and b2,j is the second column of Bj. As k → ∞, the k-period ahead forecast error covariance matrix tends
towards the unconditional covariance matrix of qt.
The forecast error variance of q1t attributable to the orthogonalized innovations in q1t is Þrst diagonal element in the Þrst summation which
2.1. UNRESTRICTED VECTOR AUTOREGRESSIONS |
31 |
is labeled a in (2.12). The forecast error variance in q1t attributable to innovations in q2t is given by the Þrst diagonal element in the second summation (labeled b). Similarly, the second diagonal element of a is the forecast error variance in q2t attributable to innovations in q1t and the second diagonal element in b is the forecast error variance in q2t attributable to innovations in itself.
A problem you may encountered in practice is that the forecast error decomposition and impulse responses may be sensitive to the ordering of the variables in the orthogonalizing process, so it may be a good idea to experiment with which variable is q1t and which one is q2t. A second problem is that the procedures outlined above are purely of a statistical nature and have little or no economic content. In chapter (8.4) we will cover a popular method for using economic theory to identify the shocks.
Potential Pitfalls of Unrestricted VARs
Cooley and LeRoy [32] criticize unrestricted VAR accounting because the statistical concepts of Granger causality and econometric exogeneity are very di erent from standard notions of economic exogeneity. Their point is that the unrestricted VAR is the reduced form of some structural model from which it is not possible to discover the true relations of cause and e ect. Impulse response analyses from unrestricted VARs do not necessarily tell us anything about the e ect of policy interventions on the economy. In order to deduce cause and e ect, you need to make explicit assumptions about the underlying economic environment.
We present the Cooley—LeRoy critique in terms of the two-equation model consisting of the money supply and the nominal exchange rate
m |
= ²1, |
|
|
|
|
|
(2.13) |
s |
= γm + ²2, |
|
|
|
|
(2.14) |
|
where the error terms are related by ² |
= λ² |
+ ² |
|
with ² |
iid |
N(0, σ2), |
|
iid |
2 |
1 |
|
3 |
1 |
|
1 |
²3 N(0, σ32) and E(²1²3) = 0. Then you can rewrite (2.13) and (2.14) |
|||||||
as |
|
|
|
|
|
|
|
m = |
²1, |
|
|
|
|
|
(2.15) |

32 CHAPTER 2. SOME USEFUL TIME-SERIES METHODS
s = γm + λ²1 + ²3. |
(2.16) |
m is exogenous in the economic sense and m = ²1 determines part of ²2. The e ect of a change of money on the exchange rate ds = (λ + γ)dm is well deÞned.
A reversal of the causal link gets you into trouble because you will not be able to unambiguously determine the e ect of an m shock on s. Suppose that instead of (2.13), the money supply is governed by
|
|
iid |
iid |
two components, ²1 = δ²2 + ²4 with ²2 N(0, σ22), ²4 |
N(0, σ42) and |
||
E(²4²2) = 0. Then |
|
|
|
m |
= |
δ²2 + ²4, |
(2.17) |
s |
= |
γm + ²2. |
(2.18) |
If the shock to m originates with ²4, the e ect on the exchange rate is ds = γd²4. If the m shock originates with ²2, then the e ect is ds = (1 + γδ)d²2.
Things get really confusing if the monetary authorities follow a feedback rule that depends on the exchange rate,
m |
= |
θs + ²1, |
(2.19) |
s |
= |
γm + ²2, |
(2.20) |
where E(²1²2) = 0. The reduced form is
m |
= |
²1 + θ²2 |
, |
(2.21) |
||
1 − γθ |
||||||
|
|
|
|
|
||
s |
= |
γ²1 + ²2 |
. |
(2.22) |
||
|
||||||
|
|
1 − γθ |
|
|
|
Again, you cannot use the reduced form to unambiguously determine the e ect of m on s because the m shock may have originated with ²1, ²2, or some combination of the two. The best you can do in this case is to run the regression s = βm + η, and get β = Cov(s, m)/Var(m) which is a function of the population moments of the joint probability distribution for m and s. If the observations are normally distributed, then E(s|m) = βm, so you learn something about the conditional expectation of s given m. But you have not learned anything about the e ects of policy intervention.
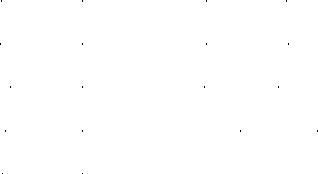
2.1. UNRESTRICTED VECTOR AUTOREGRESSIONS |
33 |
To relate these ideas to unrestricted VARs, consider the dynamic model
mt |
= |
θst + β11mt−1 + β12st−1 + ²1t, |
(2.23) |
st |
= |
γmt + β21mt−1 + β22st−1 + ²2t, |
(2.24) |
iid |
|
iid |
|
where ²1t N(0, σ12), ²2t N(0, σ22), and E(²1t²2s) = 0 for all t, s.
Without additional restrictions, ²1t and ²2t are exogenous but both mt and st are endogenous. Notice also that mt−1 and st−1 are exogenous with respect to the current values mt and st.
If θ = 0, then mt is said to be econometrically exogenous with respect to st. mt, mt−1, st−1 would be predetermined in the sense that an intervention due to a shock to mt can unambiguously be attributed to ²1t and the e ect on the current exchange rate is dst = γdmt. If β12 = θ = 0, then mt is strictly exogenous to st.
Eliminate the current value observations from the right side of (2.23)
and (2.24) to get the reduced form |
|
|
|
|
|
|
|
|
|
|||||||
mt |
= π11mt−1 + π12st−1 + umt, |
|
(2.25) |
|||||||||||||
|
st |
= π21mt−1 + π22st−1 + ust, |
|
(2.26) |
||||||||||||
where |
|
|
|
|
|
|
|
|
|
|
|
|
|
|
|
|
|
(β11 + θβ21) |
|
|
|
(β12 + θβ22) |
|
||||||||||
π11 = |
|
|
|
|
|
, |
π12 = |
|
|
|
|
, |
|
|||
|
|
|
|
(1 − γθ) |
|
(1 − γθ) |
|
|
||||||||
π21 = |
(β21 + γβ11) |
, |
π22 = |
|
(β22 + γβ12) |
|
||||||||||
|
|
|
(1 − γθ) |
|
(1 − γθ) |
|
|
|
|
|||||||
umt = |
(²1t + θ²2t) |
, |
ust = |
(²2t + γ²1t) |
, |
|
|
|
||||||||
|
(1 − γθ) |
|
(1 − γθ) |
|
|
|
||||||||||
|
|
|
(σ2 + θ2σ2) |
|
|
|
|
(γ2σ2 |
+ σ2) |
|
||||||
Var(umt) = |
|
|
|
|
1 |
2 |
, |
Var(ust) = |
|
1 |
2 |
, |
||||
|
|
|
(1 − γθ)2 |
|
− γθ)2 |
|||||||||||
|
|
|
|
|
|
(1 |
|
|||||||||
|
|
(γσ2 |
+ θσ2) |
|
|
|
|
|
|
|
|
|
|
|||
Cov(umt, ust) = |
|
|
|
|
1 |
2 |
. |
|
|
|
|
|
|
|
|
|
|
|
|
(1 − γθ)2 |
|
|
|
|
|
|
|
|
|
||||
|
|
|
|
|
|
|
|
|
|
|
|
|
|
(14) (last 3 If you were to apply the VAR methodology to this system, you expressions)
would estimate the π coe cients. If you determined that π12 = 0,
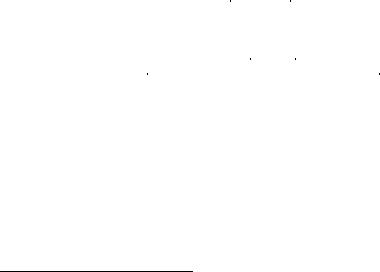
34 CHAPTER 2. SOME USEFUL TIME-SERIES METHODS
you would say that s does not Granger cause m (and therefore m is econometrically exogenous to s). But when you look at (2.23) and (2.24), m is exogenous in the structural or economic sense when θ = 0 but this is not implied by π12 = 0. The failure of s to Granger cause m need not tell us anything about structural exogeneity.
Suppose you orthogonalize the error terms in the VAR. Let δ = Cov(umt, ust)/Var(umt) be the slope coe cient from the linear projection of ust onto umt. Then ust − δumt is orthogonal to umt by construction. An orthogonalized system is obtained by multiplying (2.25) by δ and subtracting this result from (2.26)
mt = π11mt−1 + π12st−1 + umt, |
(2.27) |
st = δmt + (π21 − δπ11)mt−1 + (π22 − δπ12)st−1 + ust − δumt. |
(2.28) |
The orthogonalized system includes a current value of mt in the st equation but it does not recover the structure of (2.23) and (2.24). The orthogonalized innovations are
|
|
|
umt = |
²1t + θ²2t |
, |
|
|
(2.29) |
|||
|
|
|
1 − γθ |
|
|
||||||
|
|
|
(γ²1t + ²2t) − ³ |
γσ2 |
+θσ2 |
´ (²1t + θ²2t) |
|
|
|||
|
|
|
1 |
2 |
|
|
|
||||
ust |
− |
δumt = |
σ12+θ2σ22 |
|
, |
(2.30) |
|||||
|
− |
γθ |
|
|
|
||||||
|
|
|
1 |
|
|
|
|
|
|||
|
|
|
|
|
|
|
|
|
which allows you to look at shocks that are unambiguously attributable to umt in an impulse response analysis but the shock is not unambiguously attributable to the structural innovation, ²1t.
To summarize, impulse response analysis of unrestricted VARs provide summaries of dynamic correlations between variables but correlations do not imply causality. In order to make structural interpretations, you need to make assumptions of the economic environment and build them into the econometric model.6
6You’ve no doubt heard the phrase made famous by Milton Friedman, “There’s no such thing as a free lunch.” Michael Mussa’s paraphrasing of that principle in doing economics is “If you don’t make assumptions, you don’t get conclusions.”