
- •Preface
- •Contents
- •Chapter 1
- •1.1 International Financial Markets
- •Foreign Exchange
- •Covered Interest Parity
- •Uncovered Interest Parity
- •Futures Contracts
- •1.2 National Accounting Relations
- •National Income Accounting
- •The Balance of Payments
- •1.3 The Central Bank’s Balance Sheet
- •Chapter 2
- •2.1 Unrestricted Vector Autoregressions
- •Lag-Length Determination
- •Granger Causality, Econometric Exogeniety and Causal
- •Priority
- •The Vector Moving-Average Representation
- •Impulse Response Analysis
- •Forecast-Error Variance Decomposition
- •Potential Pitfalls of Unrestricted VARs
- •2.2 Generalized Method of Moments
- •2.3 Simulated Method of Moments
- •2.4 Unit Roots
- •The Levin—Lin Test
- •The Im, Pesaran and Shin Test
- •The Maddala and Wu Test
- •Potential Pitfalls of Panel Unit-Root Tests
- •2.6 Cointegration
- •The Vector Error-Correction Representation
- •2.7 Filtering
- •The Spectral Representation of a Time Series
- •Linear Filters
- •The Hodrick—Prescott Filter
- •Chapter 3
- •The Monetary Model
- •Cassel’s Approach
- •The Commodity-Arbitrage Approach
- •3.5 Testing Monetary Model Predictions
- •MacDonald and Taylor’s Test
- •Problems
- •Chapter 4
- •The Lucas Model
- •4.1 The Barter Economy
- •4.2 The One-Money Monetary Economy
- •4.4 Introduction to the Calibration Method
- •4.5 Calibrating the Lucas Model
- •Appendix—Markov Chains
- •Problems
- •Chapter 5
- •Measurement
- •5.2 Calibrating a Two-Country Model
- •Measurement
- •The Two-Country Model
- •Simulating the Two-Country Model
- •Chapter 6
- •6.1 Deviations From UIP
- •Hansen and Hodrick’s Tests of UIP
- •Fama Decomposition Regressions
- •Estimating pt
- •6.2 Rational Risk Premia
- •6.3 Testing Euler Equations
- •Volatility Bounds
- •6.4 Apparent Violations of Rationality
- •6.5 The ‘Peso Problem’
- •Lewis’s ‘Peso-Problem’ with Bayesian Learning
- •6.6 Noise-Traders
- •Problems
- •Chapter 7
- •The Real Exchange Rate
- •7.1 Some Preliminary Issues
- •7.2 Deviations from the Law-Of-One Price
- •The Balassa—Samuelson Model
- •Size Distortion in Unit-Root Tests
- •Problems
- •Chapter 8
- •The Mundell-Fleming Model
- •Steady-State Equilibrium
- •Exchange rate dynamics
- •8.3 A Stochastic Mundell—Fleming Model
- •8.4 VAR analysis of Mundell—Fleming
- •The Eichenbaum and Evans VAR
- •Clarida-Gali Structural VAR
- •Appendix: Solving the Dornbusch Model
- •Problems
- •Chapter 9
- •9.1 The Redux Model
- •9.2 Pricing to Market
- •Full Pricing-To-Market
- •Problems
- •Chapter 10
- •Target-Zone Models
- •10.1 Fundamentals of Stochastic Calculus
- •Ito’s Lemma
- •10.3 InÞnitesimal Marginal Intervention
- •Estimating and Testing the Krugman Model
- •10.4 Discrete Intervention
- •10.5 Eventual Collapse
- •Chapter 11
- •Balance of Payments Crises
- •Flood—Garber Deterministic Crises
- •11.2 A Second Generation Model
- •Obstfeld’s Multiple Devaluation Threshold Model
- •Bibliography
- •Author Index
- •Subject Index
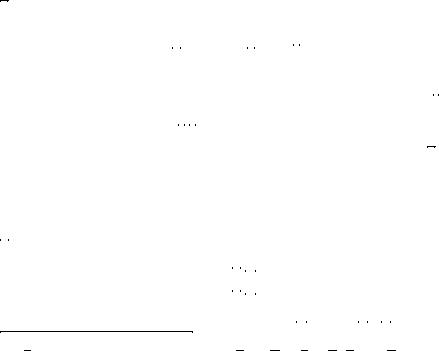
|
24 |
|
CHAPTER 2. SOME USEFUL TIME-SERIES METHODS |
||||||
|
independently and identically distributed according to some unspeci- |
||||||||
|
|
|
|
|
|
|
|
|
D |
|
Þed distribution with mean µ and variance σ2, YT → N(µ, σ2) indicates |
||||||||
|
that as T → ∞, the sequence of random variables YT2 converges in dis- |
||||||||
|
tribution |
to the normal with mean µ and variance σ and is called the |
|||||||
|
asymptotic distribution of YT . This means that for su ciently large T , |
||||||||
|
the |
random variable |
{ |
Y |
T } |
has the normal distribution with mean µ and |
|||
(3) |
|
2 |
|
|
|
|
|||
variance σ |
. We will say that a time-series {xt} is covariance station- |
||||||||
|
ary if its Þrst and second moments are Þnite and are time invariant—for |
||||||||
|
example, if E(xt) = |
µ, and E(xtxt−j) = γj. AR(p) stands for au- |
|||||||
|
toregression |
of order p, MA(n) stands for moving average of order |
n, ARIMA stands for autoregressive-integrated-moving-average, VAR stands for vector autoregression, and VECM stands for vector error correction model.
2.1Unrestricted Vector Autoregressions
Consider a zero-mean covariance stationary bivariate vector time-series, qt = (q1t, q2t)0 and assume that it has the p-th order autoregressive representation2
p
X
qt = Ajqt−j + ²t, |
(2.1) |
j=1
Ã!
where Aj |
= |
a11,j |
a12,j |
and the error vector has mean, E(²t) = 0 |
|
a21,j |
a22,j |
||||
|
|
|
and covariance matrix E(²t²0t) = Σ. The unrestricted vector autoregression VAR is a statistical model for the vector time-series qt. The same variables appear in each equation as the independent variables so the VAR can be e ciently estimated by running least squares (OLS) individually on each equation.
To estimate a p−th order VAR for this 2−equation system, let z0t = (q1t−1, . . . , q1t−p, q2t−1, . . . , q2t−p) and write (2.1) out as
|
|
q1t |
= z0 |
β |
1 |
+ ²1t, |
|
|
|
|
|
t |
|
|
|
|
|
|
|
q2t |
= z0 |
β |
|
+ ²2t. |
|
|
|
|
|
t |
|
2 |
|
|
|
|
|
|
|
|
|
|||
(4) |
Let the grand coe cient |
vector be β |
1 |
2 |
||||
|
= (β0 |
, β0 )0, and let |
2qt will be covariance stationary if E(qt) = µ, E(qt − µ)(qt−j − µ)0 = Σj.
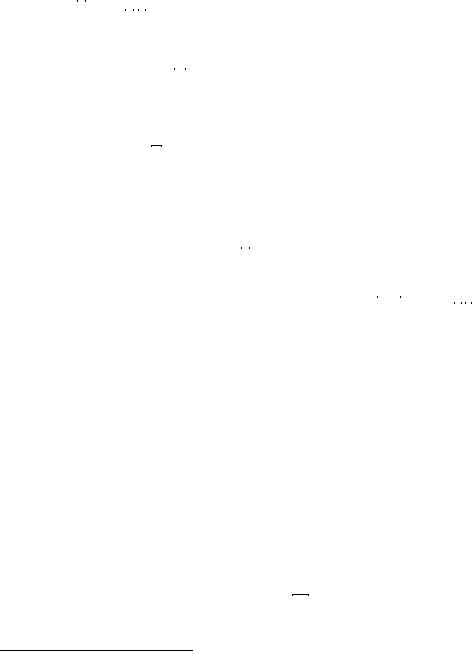
2.1. UNRESTRICTED VECTOR AUTOREGRESSIONS |
25 |
|
|
|||||||||||||
Q = plim |
|
1 |
|
T |
q |
q0 , be a positive deÞnite matrix of constants which |
|
(5) |
||||||||
T |
t=1 |
|||||||||||||||
|
|
|
t |
|
t |
|
|
|
|
|||||||
|
|
|
|
|
|
|
|
|||||||||
exists by |
the law of large numbers and the covariance stationarity as- |
|
|
|||||||||||||
³ P |
|
|
|
´ |
|
|
|
|
|
|
|
|||||
sumption. Then, as T → ∞ |
|
|
|
|
|
|||||||||||
|
|
|
|
|
|
|
|
√ |
|
|
ˆ |
D |
(2.2) |
|
|
|
|
|
|
|
|
|
|
|
|
|
|
||||||
|
|
|
|
|
|
|
|
|
T (β |
− β) → N(0, Ω), |
|
|
where Ω = Σ Q−1. The asymptotic distribution can be used to test (6) hypotheses about the β vector.
Lag-Length Determination
Unless you have a good reason to do otherwise, you should let the data determine the lag length p. If the qt are drawn from a normal distri-
|
the log likelihood function for (2.1) is |
2 ln Σ + c where c is a |
|||||||
bution, |
3 |
− |
| |
| |
|
|
|
|
|
constant. If you choose the lag-length to maximize the normal likeli- |
|||||||||
|
ˆ |
|
ˆ |
|
1 |
|
|
T |
0 |
hood you just choose p to minimize ln |Σp|, where |
Σp |
= |
T −p |
|
|
t=p+1 |
ˆ²tˆ² t |
||
is the estimated error covariance matrix of the VAR(p). In |
applications |
||||||||
|
P |
|
|
with sample sizes typically available to international macroeconomists— 100 or so quarterly observations—using the likelihood criterion typically results in choosing ps that are too large. To correct for the upward small-sample bias, two popular information criteria are frequently used for data-based lag-length determination. They are AIC suggested by Akaike [1], and BIC suggested by Schwarz [125]. Both AIC and BIC modify the likelihood by attaching a penalty for adding additional lags.
Let k be the total number of regression coe cients (the aij,r coefÞcients in (2.1)) in the system. In our bivariate case k = 4p.4 The log-likelihood cannot decrease when additional regressors are included. Akaike [1] proposed attaching a penalty to the likelihood for adding lags and to choose p to minimize
AIC = 2 ln |Σˆp| + 2Tk.
3|Σ| denotes the determinant of the matrix Σ.
4This is without constants in the regressions. If constants are included in the VAR then k = 4p + 2.
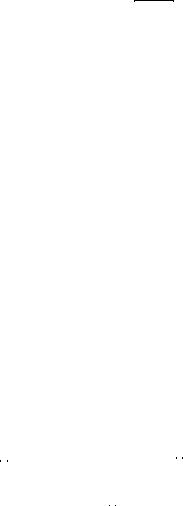
26 CHAPTER 2. SOME USEFUL TIME-SERIES METHODS
Even with the penalty, AIC often suggests p to be too large. An alternative criterion, suggested by Schwarz [125] imposes an even greater penalty for additional parameters is
BIC = 2 ln |Σˆp| + |
k ln T |
|
T . |
(2.3) |
Granger Causality, Econometric Exogeniety and Causal
Priority
In VAR analysis, we say q1t does not Granger cause q2t if lagged q1t do not appear in the equation for q2t. That is, conditional upon current and lagged q2t, current and lagged q1t do not help to predict future q2t. You can test the null hypothesis that q1t does not Granger cause q2t by regressing q2t on lagged q1t and lagged q2t and doing an F-test for the joint signiÞcance of the coe cients on lagged q1t.
If q1t does not Granger cause q2t, we say q2t is econometrically exogenous with respect to q1t. If it is also true that q2t does Granger cause q1t, we say that q2t is causally prior to q1t.
The Vector Moving-Average Representation
Given the lag length p, you can estimate invert the VAR(p) to get the Wold vector
the Aj coe cients by OLS and moving-average representation
q |
t = |
I − p |
AjLj |
−1 |
²t |
|
|
|
|
jX |
|
|
|
|
|
|
=1 |
|
|
|
|
|
∞ |
|
|
|
|
= |
jX |
CjLj²t, |
|
(2.4) |
||
|
|
|||||
|
|
=0 |
|
|
|
|
where L is the lag operator such that Ljxt = xt−j for any variable xt. To solve for the Cj matrices, you equating coe cients on powers of the lag operator. From (2.4) you know that (P∞j=0 CjLj)(I − Ppj=1 AjLj) = I.
2.1. UNRESTRICTED VECTOR AUTOREGRESSIONS |
27 |
Write it out as
I= C0 + (C1 − C0A1)L + (C2 − C1A1 − C0A2)L2 +(C3 − C2A1 − C1A2 − C0A3)L3
+(C4 − C3A1 − C2A2 − C1A3 − C0A4)L4 + · · ·
=X∞ Cj − Xj Cj−kAk Lj.
j=0 k=1
Now to equate coe cients on powers of L, Þrst note that C0 = I and the rest of the Cj follow recursively
C1 = A1,
C2 |
= C1A1 + A2, |
|
C3 |
= C2A1 + C1A2 + A3, |
|
C4 |
= C3A1 + C2A2 + C1A3 + A4, |
|
|
. |
|
|
. |
|
|
. |
|
|
|
k |
Ck |
|
jX |
= |
Ck−jAj. |
|
|
|
=1 |
(7) (see line 2)
(8)(formulae to end of section)
For example if p = 2, set Aj = 0 for j ≥ 3. Then C1 = A1, C2 |
= |
C1A1 + A2, C3 = C2A1 + C1A2, C4 = C3A1 + C2A2, and so on. |
(9) |
Impulse Response Analysis
Once you get the moving-average representation you will want employ impulse response analysis to evaluate the dynamic e ect of innovations in each of the variables on (q1t, q2t). When you go to simulate the dynamic response of q1t and q2t to a shock to ²1t, you are immediately
confronted with two problems. The Þrst one is how big should the (10) shock be? This becomes an issue because you will want to compare the response of q1t across di erent shocks. You’ll have to make a normalization for the size of the shocks and a popular choice is to consider
shocks one standard deviation in size. The second problem is to get shocks that can be unambiguously attributed to q1t and to q2t. If ²1t and ²2t are contemporaneously correlated, however, you can’t just shock ²1t and hold ²2t constant.
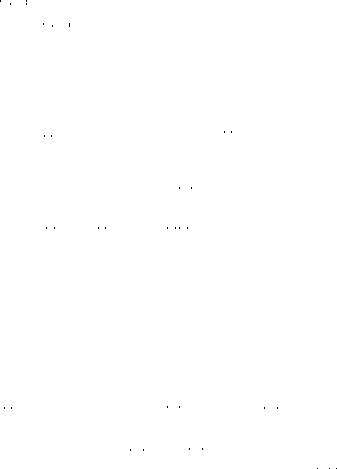
28 CHAPTER 2. SOME USEFUL TIME-SERIES METHODS
To deal with these problems, Þrst standardize the innovations. Since the correlation matrix is given by
|
|
|
|
|
R = ΛΣΛ = |
à ρ |
1 |
! , |
||
|
|
|
|
|
|
|
1 |
ρ |
|
|
|
|
1 |
0 |
|
|
|
|
|||
|
|
|
|
|
|
|
|
|||
where Λ = |
√σ11 |
is a matrix with the inverse of the standard |
||||||||
1 |
||||||||||
|
|
0 |
√ |
|
|
|
|
|
||
|
σ22 |
|
|
|
deviations on the diagonal and zeros elsewhere. The error covariance matrix can be decomposed as Σ = Λ−1RΛ−1. This means the Wold vector moving-average representation (2.4) can be re-written as
X∞
qt =
j=0
= X∞
CjLj |
|
Λ−1 |
(Λ²t) |
|
|
|
|
|
|
|
(2.5) |
DjLj vt. |
j=0
where Dj ≡ CjΛ−1,vt ≡ Λ²t and E(vtv0t) = R. The newly deÞned innovations v1t and v2t both have variance of 1.
Now to unambiguously attribute an innovation to q1t, you must orthogonalize the innovations by taking the unique upper triangular Choleski matrix decomposition of the correlation matrix R = S0S,
where S = Ã |
s11 |
s12 |
! . Now insert SS−1 into the normalized moving |
||||||||||
0 |
|
s22 |
|||||||||||
average (2.5) to get |
|
|
S S−1vt |
|
|
|
|
|
|
||||
|
|
qt |
= ∞ |
DjLj |
= |
∞ |
BjLjηt, |
(2.6) |
|||||
|
|
|
|
X |
|
|
|
X |
|
|
|
|
|
|
|
|
|
|
j=0 |
|
³ |
´ |
j=0 |
|
|
|
|
(11) (eq. 2.6) where Bj ≡ DjS = CjΛ−1S and ηt ≡ S−1vt, is the 2×1 vector of zero- |
|||||
mean orthogonalized innovations with covariance matrix E( |
ηtη |
t0 = I). |
|||
Note that S−1 is also upper triangular. |
|
|
|
||
Now write out the individual equations in (2.6) to get |
|
|
|||
|
|
∞ |
∞ |
|
|
q1t |
|
jX |
X |
|
|
= |
b11,jη1,t−j + |
b12,jη2,t−j, |
(2.7) |
||
|
|
=0 |
j=0 |
|
|
|
|
∞ |
∞ |
|
|
|
|
jX |
X |
|
|
q2t |
= |
b21,jη1,t−j + |
b22,jη2,t−j. |
(2.8) |
|
|
|
=0 |
j=0 |
|
|